My family and I just got back from a India vacation. As always, we had a great time and as always, the travel was painful. One, because of its length and also because of all the documentation checks at various points in the journey. But in hindsight, I am feeling thankful that we were back in the States before the latest terrorist attack on the NWA jetliner to Detroit took place. A Nigerian man, Umar Farouk AbdulMutallak, tried to set off an explosive device but thankfully did not succeed.
Now apparently, this individual was on the anti-terrorism radar for a while. He was on the terrorist watch-list but not on the official no-fly list. Hence, he was allowed to board the flight going from Amsterdam to Detroit, where he tried to perpetrate his misdeed. The events have raised a number of valid questions on the job the TSA (the agency in charge of ensuring safe air travel within and to/from the US) is doing in spotting these kinds of threats. There were a number of red flags in this case. A passenger who had visited Yemen - a place as bad as Pakistan when it comes to providing a safe haven for terrorists. A ticket paid in cash. Just one carry-on bag and no bags checked in. A warning coming from this individual's family, no less. A denied British visa - another country that has as much to fear from terrorism as the US. The question I have is: could more have been done? Could analytics have been deployed more effectively to identify and isolate the perpetrator? And how could all of this be achieved without giving a very overt impression of profiling? A few ideas come to mind.
First, a scoring system to constantly upgrade the threat level of individuals and provide a greater amount of precision in understanding the threat posed by an individual at a certain point in time. A terror list of 555,000 is too bloated and is likely to contain a fair number of false positives. This model would use latest information about the traveler, all of which can be gathered at the time of travel or before travel. Is the traveler a US citizen or a citizen of a friendly country? (US Citizen or Perm Resident = 1, Citizen of US ally = 2, Other countries = 3, Known terrorist nation = 5) Has the person bought the ticket in cash or by electronic payment? (Electronic payment = 1, Physical instrument such as a cheque = 2, Cash = 5) Does the person have a US contact? Is the contact a US citizen or a permanent resident? Is the person traveling to a valid residential address? What are the countries the individual has visited in the last 24 months? And so on. You get the idea. Now the weights that have been attached are quite arbitrary to start, but they can always be adjusted as the perception of these risk factors change and our understanding evolves.
Now what needs to be done is to update the parameters of this model every 3-6 months or so. Then every individual on the database as well as very person traveling needs to be scored using this model and high scorers (high risk of either having connections to terrorist network or traveling with some nefarious intent) can be identified for additional screening and scrutiny. These are the types of common-sense solutions that can be deployed to solve these types of ticklish problems. When the size of the problem has been reduced from 555,000 people on whom you need to spend the same amount of time, to one where the amount of scrutiny can be sloped based on the propensity to cause trouble, the problem suddenly becomes a lot more tractable.
A place where models, software engineering and the science of decision making come together to make the future a better place
Tuesday, December 29, 2009
Monday, December 28, 2009
Some end of year reading
1. Krugman on the America's own lost decade - link here
The usual Krugman rant on how things are going downhill and accelerating.
2. The Freakonomics blog on the practice of not inflation-adjusting stock returns - link here
Stock returns are seldom adjusted for inflation, transaction costs and taxes. While usually savvy investors do account for these factors, it is easy to get misled unless one reads the fine print.
3. How did buy and hold do in the last 10 years? link here
It is unfair asking a stock picker to pick just one stock. Makes for good headlines but does not really allow the stock picker to demonstrate their skills. The probability that any one company could be impacted by freak events is usually pretty high.
4. Health Statistics and the mammogram controversy - link here (from the WSJ) and here from the Numbers Guy blog
Reading any kind of reporting coming from the US (except sports, maybe) has become a painful drag through the ideology of the author.
5. Happiness - State of mind or state of body? - link here
An interesting 'light' piece of reading. Turns out that they are one and the same thing.
The usual Krugman rant on how things are going downhill and accelerating.
2. The Freakonomics blog on the practice of not inflation-adjusting stock returns - link here
Stock returns are seldom adjusted for inflation, transaction costs and taxes. While usually savvy investors do account for these factors, it is easy to get misled unless one reads the fine print.
3. How did buy and hold do in the last 10 years? link here
It is unfair asking a stock picker to pick just one stock. Makes for good headlines but does not really allow the stock picker to demonstrate their skills. The probability that any one company could be impacted by freak events is usually pretty high.
4. Health Statistics and the mammogram controversy - link here (from the WSJ) and here from the Numbers Guy blog
Reading any kind of reporting coming from the US (except sports, maybe) has become a painful drag through the ideology of the author.
5. Happiness - State of mind or state of body? - link here
An interesting 'light' piece of reading. Turns out that they are one and the same thing.
Labels:
Investing,
Macroeconomics,
statistical inference
Saturday, December 19, 2009
The place of Systems Modeling in Analytics
When one talks about predictive analytics, the typical thought process goes in the direction of regression, neural nets, data mining techniques. Techniques that savvy marketers (consumer product companies, banks) have been using for close to two decades now in building insights about consumer behaviour. Systems modeling or Systems Dynamics is not something that immediately springs to mind.
So what is systems modeling all about? Systems modeling is creating a mathematical representation of a real-world phenomenon, trying to cover as wide range a set of inputs as feasible and the most valuable outputs. The systems model tries to explain how the inputs translate to outputs. How the systems model is different from a statistical predictive model is that the purpose of the systems model is not to try and explain variance in the output. The systems model instead tries to establish structural relationships between the input and the output. The model then further stresses the structural relationship by varying the inputs and looking at the impact on the output.
A good example of a subject that can be systems-modeled (my verb!) is the problem of terrorism. The problem has different inputs: unhappy people, territorial disputes, foreign power wanting to create trouble, funding, media coverage, etc. The immediate output is various actions of terrorism such as assassinations of leaders, suicide bombings, etc. It might be feasible to build a model that creates a structure on how these various inputs combine and interact with one another and cause the outputs. (If one goes back over the past 150 years, there should be plenty of data points.) Another way of looking at the output is a more holistic view that measures the damage done in terms of lives lost, economic damage incurred, etc.
What would be the purposes of this model? In my opinion, the value of such a model is less around where the next terrorist strike is going to be, or how big the next strike is going to be. (This is incidentally what a classic statistical model is going to try to do.) But rather, the model should try and explain what are the confluence of factors that produce a large output event (lives lost, economic damage) and how can some of the factors be controlled, ONCE an insurgency is already underway. The hypothetical model I am talking about does not try to predict, but rather to strengthen our understanding of the system dynamics. The model would have a PoV on what inputs can be controlled and to what extent are they controllable.
The model would then be used to understand how a large impact event can be prevented or its impact minimized. So if the federal government had a $100 billion to spend, how much should they spend on homeland security vs. promoting a positive image of the United States through foreign media? The model might tell that it is pointless to spend more than, say, $500 million on putting in a sophisticated software to block large untraced wire transfers as there are other ways in which the funding can be made available to the perpetrators of the terrorism act. So controlling the funding for an insurgency through sophisticated money laundering and layering detection algorithms may be pointless if the actual money gets exchanged through a non-electronic channel.
So an agency interested in curbing terrorism, might be better advised in, say, over-investing in trauma care health facilities and emergency services in vulnerable areas. This is so that when a strike does take place, medical help for the people who are affected is close at hand and casualties are minimized.
Why am I writing all this? Analytical problem solving is not just about fancy statistical algorithms or cool math, it is also about thinking hard about problems and creating their mathematical representations - and then being crystal clear about what those mathematical representations can and cannot do. This is where the systems modeling approach can be a very effective portion of the arsenal of a business modeler.
So what is systems modeling all about? Systems modeling is creating a mathematical representation of a real-world phenomenon, trying to cover as wide range a set of inputs as feasible and the most valuable outputs. The systems model tries to explain how the inputs translate to outputs. How the systems model is different from a statistical predictive model is that the purpose of the systems model is not to try and explain variance in the output. The systems model instead tries to establish structural relationships between the input and the output. The model then further stresses the structural relationship by varying the inputs and looking at the impact on the output.
A good example of a subject that can be systems-modeled (my verb!) is the problem of terrorism. The problem has different inputs: unhappy people, territorial disputes, foreign power wanting to create trouble, funding, media coverage, etc. The immediate output is various actions of terrorism such as assassinations of leaders, suicide bombings, etc. It might be feasible to build a model that creates a structure on how these various inputs combine and interact with one another and cause the outputs. (If one goes back over the past 150 years, there should be plenty of data points.) Another way of looking at the output is a more holistic view that measures the damage done in terms of lives lost, economic damage incurred, etc.
What would be the purposes of this model? In my opinion, the value of such a model is less around where the next terrorist strike is going to be, or how big the next strike is going to be. (This is incidentally what a classic statistical model is going to try to do.) But rather, the model should try and explain what are the confluence of factors that produce a large output event (lives lost, economic damage) and how can some of the factors be controlled, ONCE an insurgency is already underway. The hypothetical model I am talking about does not try to predict, but rather to strengthen our understanding of the system dynamics. The model would have a PoV on what inputs can be controlled and to what extent are they controllable.
The model would then be used to understand how a large impact event can be prevented or its impact minimized. So if the federal government had a $100 billion to spend, how much should they spend on homeland security vs. promoting a positive image of the United States through foreign media? The model might tell that it is pointless to spend more than, say, $500 million on putting in a sophisticated software to block large untraced wire transfers as there are other ways in which the funding can be made available to the perpetrators of the terrorism act. So controlling the funding for an insurgency through sophisticated money laundering and layering detection algorithms may be pointless if the actual money gets exchanged through a non-electronic channel.
So an agency interested in curbing terrorism, might be better advised in, say, over-investing in trauma care health facilities and emergency services in vulnerable areas. This is so that when a strike does take place, medical help for the people who are affected is close at hand and casualties are minimized.
Why am I writing all this? Analytical problem solving is not just about fancy statistical algorithms or cool math, it is also about thinking hard about problems and creating their mathematical representations - and then being crystal clear about what those mathematical representations can and cannot do. This is where the systems modeling approach can be a very effective portion of the arsenal of a business modeler.
I'll close out with a couple of links, which prompted this wave of thinking on this subject. One is a paper in the Nature journal where the authors have presented a statistical model of insurgency events. The link is here. It's a gated article.
The following link has a very good critique on the article.
Monday, December 14, 2009
The new lean economy
I have commented earlier (link here) on the phenomenon of the jobless recovery that the US economy (and definitely many of the more 'open' economies) are likely to be facing in the next few years. Of course, this precludes any serious effort by the government to create jobs through stimulus like efforts - though there is going to be a limit to that as well, given the relation of stimulus efforts to future debt creation.
In my opinion, the 2008-09 Great Recession has forced companies to seriously evaluate their cost structures. A lot of what passed before has been cut (in the fat bubble years) and companies have begun to realize serious benefits from cutting out fat, leveraging efficiencies at the work place by eliminating redundancies, moving their applications to open source platforms and so on. And my intuition is that many of these changes are not going to be just a reaction to the downturn. Companies are seeing that the quality of output has not significantly suffered because there aren't enough people to do the work, or because the work is no longer being done by expensive software. Thomas Friedman, in a piece in the New York Times, wrote that the Great Recession has also brought about a Great Inflection.
According to Friedman, the Great Inflection is "the mass diffusion of low-cost, high-powered innovation technologies — from hand-held computers to Web sites that offer any imaginable service — plus cheap connectivity. They are transforming how business is done." Friedman talks about two examples in his piece. One is a small, not-for-profit that needs to create an ad campaign. Given constrained budgets, the need of the hour is to be innovative, but with cost efficiencies firmly in mind. The ad creator uses a mix of collaboration tools (enabled by cheap and high throughput communication), online sourcing (through the availability of online marketplaces for media products) and multimedia editing (enabled by software and hardware improvements) to deliver a solution that is both innovative and appealing as well as one that fits in the client budget.
The second example, of the furniture manufacturer Ethan Allen, talks about transformations the business has had to make to drive productivity improvements. The transformations have been both traditional: workforce reductions of over 25%, multiskilling of the remaining workers to make them more fungible, consolidation of manufacturing and process engineering. Additionally the company has also adopted other non-conventional means to conserve cash and survive. This has included moving a lot of the advertising activity in-house leveraging the multimedia desktop tools that are available today.
Finally, Friedman makes a point that the flow of credit (which is still very constrained) would make these companies create jobs. I disagree. I think it is going to take a lot for companies to start hiring again. And when they do, they are going to be the multiskilled talent that is now constituting the workforce at Ethan Allen. Companies across the spectrum have tasted blood - of keeping productivity and output high and costs low. They are not likely to go back to being fat again anytime soon.
In Indian banks, I am seeing an increasing phenomenon in the growth of branches. Most big banks are expanding their branch networks - like HDFC Bank, ICICI Bank and even the venerable State Bank of India. But the branches increasingly are being staffed at low staff levels. Staff is usually multi-skilled. Specialists are assigned across branches and are mobile. As a customer, if you need any specialized service, the representative at the branch contacts the specialist who then makes an appointment within 24 hours. Instead of having committed staff in every branch, the staffing model comprises fungible generalists allotted to branches and shared, mobile specialists across branches.
In my opinion, the 2008-09 Great Recession has forced companies to seriously evaluate their cost structures. A lot of what passed before has been cut (in the fat bubble years) and companies have begun to realize serious benefits from cutting out fat, leveraging efficiencies at the work place by eliminating redundancies, moving their applications to open source platforms and so on. And my intuition is that many of these changes are not going to be just a reaction to the downturn. Companies are seeing that the quality of output has not significantly suffered because there aren't enough people to do the work, or because the work is no longer being done by expensive software. Thomas Friedman, in a piece in the New York Times, wrote that the Great Recession has also brought about a Great Inflection.
According to Friedman, the Great Inflection is "the mass diffusion of low-cost, high-powered innovation technologies — from hand-held computers to Web sites that offer any imaginable service — plus cheap connectivity. They are transforming how business is done." Friedman talks about two examples in his piece. One is a small, not-for-profit that needs to create an ad campaign. Given constrained budgets, the need of the hour is to be innovative, but with cost efficiencies firmly in mind. The ad creator uses a mix of collaboration tools (enabled by cheap and high throughput communication), online sourcing (through the availability of online marketplaces for media products) and multimedia editing (enabled by software and hardware improvements) to deliver a solution that is both innovative and appealing as well as one that fits in the client budget.
The second example, of the furniture manufacturer Ethan Allen, talks about transformations the business has had to make to drive productivity improvements. The transformations have been both traditional: workforce reductions of over 25%, multiskilling of the remaining workers to make them more fungible, consolidation of manufacturing and process engineering. Additionally the company has also adopted other non-conventional means to conserve cash and survive. This has included moving a lot of the advertising activity in-house leveraging the multimedia desktop tools that are available today.
Finally, Friedman makes a point that the flow of credit (which is still very constrained) would make these companies create jobs. I disagree. I think it is going to take a lot for companies to start hiring again. And when they do, they are going to be the multiskilled talent that is now constituting the workforce at Ethan Allen. Companies across the spectrum have tasted blood - of keeping productivity and output high and costs low. They are not likely to go back to being fat again anytime soon.
In Indian banks, I am seeing an increasing phenomenon in the growth of branches. Most big banks are expanding their branch networks - like HDFC Bank, ICICI Bank and even the venerable State Bank of India. But the branches increasingly are being staffed at low staff levels. Staff is usually multi-skilled. Specialists are assigned across branches and are mobile. As a customer, if you need any specialized service, the representative at the branch contacts the specialist who then makes an appointment within 24 hours. Instead of having committed staff in every branch, the staffing model comprises fungible generalists allotted to branches and shared, mobile specialists across branches.
Tuesday, December 8, 2009
Too Big To Fail - contd.
I have commented earlier on the TBTF doctrine.
Recently, I came across a couple of other references on the TBTF situation and what to do about it. The first from the FT has the author Willem Buiter presenting a slew of solutions on what to do about banks becoming TBTF. (Interesting how this abbreviation seems to have taken on a life of its own!) Definitely worth taking a more detailed read as the author goes to a fair degree of detail on what are some of the probable solutions.
The second is a novel way of valuing the benefit that banks get from becoming TBTF. The approach (proposed by a couple of economists Elijah Brewer and Julapa Jagtiani from the Philadelphia Fed) argues that the measure of the benefit that banks expected to get could be ascertained by the acquisition premium paid by these very banks along their journey to becoming TBTF. The estimate of this premium (looking at acquisitions from 1991 to 2004) is about $14 billion. This link references the actual paper written by the economists.
Given my obsession on getting to an optimal risk management framework for financial institutions, I thought I'd share a couple of these links.
Recently, I came across a couple of other references on the TBTF situation and what to do about it. The first from the FT has the author Willem Buiter presenting a slew of solutions on what to do about banks becoming TBTF. (Interesting how this abbreviation seems to have taken on a life of its own!) Definitely worth taking a more detailed read as the author goes to a fair degree of detail on what are some of the probable solutions.
The second is a novel way of valuing the benefit that banks get from becoming TBTF. The approach (proposed by a couple of economists Elijah Brewer and Julapa Jagtiani from the Philadelphia Fed) argues that the measure of the benefit that banks expected to get could be ascertained by the acquisition premium paid by these very banks along their journey to becoming TBTF. The estimate of this premium (looking at acquisitions from 1991 to 2004) is about $14 billion. This link references the actual paper written by the economists.
Given my obsession on getting to an optimal risk management framework for financial institutions, I thought I'd share a couple of these links.
Interesting reads from Dec 8
Interesting reads from the Net
1. Consumer credit declines for the 9th straight month - link
2. NY Fed remarks on lessons from the crisis - link
3. Credit/ Leverage and its role in creating financial crises over the years - link
I particularly liked this excerpt:
Long-run historical evidence therefore suggests that credit has an important role to play in central bank policy. Its exact role remains open to debate. After their recent misjudgements, central banks should clearly pay some attention to credit aggregates and not confine themselves simply to following targeting rules based on output and inflation.
4. The Simpson' paradox always fascinates me. This example uses unemployment rate comparisons between today and the 1981 recession - link
4b. This response by Andrew Gelman talks about when the comparison at the sub-group level is appropriate (when the definitions of the sub-groups being compared between the two samples are robust and more apples-to-apples) and also where the aggregate level is more appropriate (where the definitions have not remained stable - typically happens when the two samples are temporally divided - and therefore any comparison is not necessarily apples-to-apples) - link
Holidaying in India and just beginning to recover from the sensory overload (of family, friends, food, the media, the general environment). Really looking forward to the remaining two weeks.
1. Consumer credit declines for the 9th straight month - link
2. NY Fed remarks on lessons from the crisis - link
3. Credit/ Leverage and its role in creating financial crises over the years - link
I particularly liked this excerpt:
Long-run historical evidence therefore suggests that credit has an important role to play in central bank policy. Its exact role remains open to debate. After their recent misjudgements, central banks should clearly pay some attention to credit aggregates and not confine themselves simply to following targeting rules based on output and inflation.
4. The Simpson' paradox always fascinates me. This example uses unemployment rate comparisons between today and the 1981 recession - link
4b. This response by Andrew Gelman talks about when the comparison at the sub-group level is appropriate (when the definitions of the sub-groups being compared between the two samples are robust and more apples-to-apples) and also where the aggregate level is more appropriate (where the definitions have not remained stable - typically happens when the two samples are temporally divided - and therefore any comparison is not necessarily apples-to-apples) - link
Holidaying in India and just beginning to recover from the sensory overload (of family, friends, food, the media, the general environment). Really looking forward to the remaining two weeks.
Tuesday, November 24, 2009
Too Big To Fail or Too Scared To Confront?
Back to the blog after a long break. I do need to find a way to become more regular at updating the blog and keeping at expressing my thoughts and ideas.
What spurred this latest post was a decent article I read on the Too-Big-To-Fail (TBTF) doctrine. Of course, one is talking about banks. The article goes into details about the cost of propping up the banks and some of the estimates are truly mind-blogging.
According to the Bank of England, governments and central banks in the US, Britain, and Europe have spent or committed more than $14 trillion—the equivalent to roughly 25 per cent of the world’s economic output—to prop up financial institutions. Combined with a global recession, this bailout has undermined the public finances of the developed world.
Another related set of articles is here from the Free Exchange blog of the Economist. Raghuram Rajan from the University of Chicago School of Business has contributed some good ideas and a robust discussion of the pros and cons of various options have been presented. As always, there is little effort on the part of the various contributors to synthesize a viewpoint. Rather the tendency is to point out why a specific solution presented may not work.
To a large extent, I think these ideas miss an important point. The ideas consistently treat financial institutions as rational entities, which seem to operate largely on principles of rational economics, capital theory and other such textbook ideas. The fact of the matter is that management matters. And management is a function of the human beings that take important decisions within these organizations, their incentive structures and also, more broadly, the set of values and identity that seems to motivate these human players.
And unless we all as stakeholders begin to take notice that the destiny of corporations are driven by the individuals managing those organizations, that the laws of economics are (unlike the laws of nature) created by its human players, we will continue to argue around the margins on window dressing the regulatory system and make no significant progress towards creating a more stable financial system. A stable system by definition is going to provide fewer opportunities to pursue supernormal profits. A stable system needs the suppression of that oldest of human sins, greed. Do have the courage to confront ourselves and seriously consider a slew of workable solutions to fix a broken system? Only time will tell but I am not holding my breath.
What spurred this latest post was a decent article I read on the Too-Big-To-Fail (TBTF) doctrine. Of course, one is talking about banks. The article goes into details about the cost of propping up the banks and some of the estimates are truly mind-blogging.
According to the Bank of England, governments and central banks in the US, Britain, and Europe have spent or committed more than $14 trillion—the equivalent to roughly 25 per cent of the world’s economic output—to prop up financial institutions. Combined with a global recession, this bailout has undermined the public finances of the developed world.
Another related set of articles is here from the Free Exchange blog of the Economist. Raghuram Rajan from the University of Chicago School of Business has contributed some good ideas and a robust discussion of the pros and cons of various options have been presented. As always, there is little effort on the part of the various contributors to synthesize a viewpoint. Rather the tendency is to point out why a specific solution presented may not work.
To a large extent, I think these ideas miss an important point. The ideas consistently treat financial institutions as rational entities, which seem to operate largely on principles of rational economics, capital theory and other such textbook ideas. The fact of the matter is that management matters. And management is a function of the human beings that take important decisions within these organizations, their incentive structures and also, more broadly, the set of values and identity that seems to motivate these human players.
And unless we all as stakeholders begin to take notice that the destiny of corporations are driven by the individuals managing those organizations, that the laws of economics are (unlike the laws of nature) created by its human players, we will continue to argue around the margins on window dressing the regulatory system and make no significant progress towards creating a more stable financial system. A stable system by definition is going to provide fewer opportunities to pursue supernormal profits. A stable system needs the suppression of that oldest of human sins, greed. Do have the courage to confront ourselves and seriously consider a slew of workable solutions to fix a broken system? Only time will tell but I am not holding my breath.
Sunday, September 27, 2009
The Fed's failure in end to end risk management
Another one in a series of risk management write-ups. (I guess this is becoming more and more common as this is my full-time job right now.) I came across a recent article in the Washington Post about the malpractices in lending practiced by subprime affiliates of large banks and the reluctance of the Federal Reserve to play an effective regulatory role. The article is here.
The article talks about how the Fed gradually withdrew in its regulatory responsibility on consumer finance companies as these were not "banks". The Fed reduced its oversight of these companies because it believed it did not have the right
jurisdiction to regulate these companies. This was despite a considerable amount of evidence from individuals and other watch-dog bodies that were reporting egregious practices by these institutions. Another big factor that was playing at the time was the good old "markets self-regulate" belief (I was going to say theory and I corrected myself. Maybe I should say, myth.) but I am not going to spend too much time in this post on that.
Why did the Fed turn its head away from the problem? One of my hypotheses is too much of a reliance on "literalism". The Fed chose to literally interpret its mandate of regulating banks and decided to look no further - even though there were other institutions whose practices were exactly the same as what any bank would do. Literalism is a particular problem I have observed in the US. It is the strong objection to interpret a piece of policy/ law developed years ago in line with the world today. This problem is most commonly seen with respect to the US Constitution and its various amendments. But "literalism" is a problem when it creates blind spots in end-to-end risk management and ends up threatening the viability of the corporation or, as in this case, the entire financial system. An effective risk manager is expected to be proactive in identifying gaps in the end-to-end risk management and being open to taking on more responsibility, proposing changes to the system, as needed.
The other problem was that the Fed tended to be influenced more by grand economic theories and conceptual/ philosophical frameworks and decided to discount the data coming up from the ground. According to the article, the Fed tended to discount these pieces of anecdotal evidence as their place within a broader framework or their systematic impact was well-known. This is another problem often with smart people. It is a thinking that goes: I think and talk in concepts, abstractions and theories. Therefore, I will only listen when other people talk the same way. Now this is a problem which afflicts many of us, and therefore might be even borderline acceptable in everyday life. But this is fatal in risk management, where your job is to anticipate different ways in which the system can be at risk. An effective risk manager is expected to constantly keep her radar up for pieces of information that might be contrary to a pre-existing framework and have an efficient means of investigating whether the anecdotal evidence points to any material threat.
Finally, one important lesson that is worth taking way is that when it comes to human created systems, there is no one overarching framework or "truth". Because interactions between humans and institutions created by humans are not governed by the laws of physics, there are often no absolutes in these things. Many theories or frameworks could be simultaneously true or may apply in portions of the world we are trying to understand. Depending on the prevailing conditions, one set of rules may hold. And as conditions change or as the previous framework pushes the environment to one extreme, the competing framework often becomes more relevant and appropriate to apply. It is often practical to keep one's mind open to other theories and frameworks. Ideological alignment or obsession with one "truth" system only makes one closed to other explanations or possibilities.
The article talks about how the Fed gradually withdrew in its regulatory responsibility on consumer finance companies as these were not "banks". The Fed reduced its oversight of these companies because it believed it did not have the right

Why did the Fed turn its head away from the problem? One of my hypotheses is too much of a reliance on "literalism". The Fed chose to literally interpret its mandate of regulating banks and decided to look no further - even though there were other institutions whose practices were exactly the same as what any bank would do. Literalism is a particular problem I have observed in the US. It is the strong objection to interpret a piece of policy/ law developed years ago in line with the world today. This problem is most commonly seen with respect to the US Constitution and its various amendments. But "literalism" is a problem when it creates blind spots in end-to-end risk management and ends up threatening the viability of the corporation or, as in this case, the entire financial system. An effective risk manager is expected to be proactive in identifying gaps in the end-to-end risk management and being open to taking on more responsibility, proposing changes to the system, as needed.
The other problem was that the Fed tended to be influenced more by grand economic theories and conceptual/ philosophical frameworks and decided to discount the data coming up from the ground. According to the article, the Fed tended to discount these pieces of anecdotal evidence as their place within a broader framework or their systematic impact was well-known. This is another problem often with smart people. It is a thinking that goes: I think and talk in concepts, abstractions and theories. Therefore, I will only listen when other people talk the same way. Now this is a problem which afflicts many of us, and therefore might be even borderline acceptable in everyday life. But this is fatal in risk management, where your job is to anticipate different ways in which the system can be at risk. An effective risk manager is expected to constantly keep her radar up for pieces of information that might be contrary to a pre-existing framework and have an efficient means of investigating whether the anecdotal evidence points to any material threat.
Finally, one important lesson that is worth taking way is that when it comes to human created systems, there is no one overarching framework or "truth". Because interactions between humans and institutions created by humans are not governed by the laws of physics, there are often no absolutes in these things. Many theories or frameworks could be simultaneously true or may apply in portions of the world we are trying to understand. Depending on the prevailing conditions, one set of rules may hold. And as conditions change or as the previous framework pushes the environment to one extreme, the competing framework often becomes more relevant and appropriate to apply. It is often practical to keep one's mind open to other theories and frameworks. Ideological alignment or obsession with one "truth" system only makes one closed to other explanations or possibilities.
Tuesday, September 22, 2009
Things that I am reading this morning ...
1. Seth Godin's post about building better graphs - Link here
2. Phoenix's light rail success, driven by weekend travelers. That downtown really needed some life and looks like light rail did the trick - Link here
3. A couple of exciting sounding book reviews from The Economist. These are on my library hold list - Link here
2. Phoenix's light rail success, driven by weekend travelers. That downtown really needed some life and looks like light rail did the trick - Link here
3. A couple of exciting sounding book reviews from The Economist. These are on my library hold list - Link here
Wednesday, September 16, 2009
A case study in risk management
The credit crisis of 2008, or the Great Recession as it is now famous as, has had many many books written on it. Writers from across the ideological spectrum have written about why the crisis occured and how their brand of ideology could have prevented the crisis. Which is why I was skeptical when I came across this piece which seemed to rehash the story of the collapse of Lehman. I was pleasantly surprised that this article was about one element that has been whispered off and on, but not very convincingly: about risk management based on common sense. (The reader needs to get past the title and the opening blurb, though. The title seems to suggest the credit crisis would have never taken place if Goldman Sachs hadn't spotted the game early enough. That is plain ridiculous. The leveraging of the economy + the decline in lending standards created a ticking time-bomb. But I digress.)
The article is not about having some fancy risk management metrics or why our models are wrong or why we should not trust a Ph.D that offers to build a model for you. (Of course, all of these elements contributed to why the crisis was ignored for all these years.) Instead, the article recounts a real-life meeting that took place in Goldman Sachs at the end of 2006. The meeting was convened by Goldman CFO, David Viniar, based on some seemingly innocuous happenings. The company had been losing money on its mortgage backed securities for 10 days in a row. The resulting deep-dive into the details of the trades pointed to a sense of unease about the mortgage market. Which then caused Goldman to famously back-off from the market.
I'll leave the reading to you to get more details of what happened. But some thoughts on what contribute to effective risk management practices.
- A real-life feel for the business. You can't be just into the models, you need to be savvy enough to understand how the models you build interact with the real world outside. And it is an appreciation of this interaction that cause the hairs to stand at the back of your neck when you encounter something that just doesn't seem right.
- Proper role of risk management in the decision making hierarchy. Effective risk management takes place when the risk governance has the authority to put the brakes on risk takers (i.e., the traders, in this case). In Goldman, there were a number of enablers for this type of interaction to take place effectively. Most importantly, risk management reported to the CFO, i.e. high enough in the corporate heirarchy. Second, investment decisions needed a go-ahead from both the risk takers and risk governance.
- Mutual respect between risk governance and risk takers. Goldman encourages a collaborative style of decision making. This allows multiple conflicting opinions to be present at the table. Minority opinions are encouraged and appreciated. Over time, this fosters a culture that genuinely tolerates dissonance of opinions. This also allows the CFO to be influenced by the comptroller group as much as he typically would by the trading group.
- Finally, a certain intellectual probity to acknowledge what it does not know or understand. During the meeting, the Goldman team was not able to pinpoint what their source of unease was. But they were able to honestly admit that they didn't really understand what was going on, but that it was also most appropriate to bring the ship to harbour, given their blindspot about what they didn't know. It takes courage to back-off from an investing decision, saying "I don't understand this well enough" in the alpha-male investment banking culture.
All in all, a really interesting read.
The article is not about having some fancy risk management metrics or why our models are wrong or why we should not trust a Ph.D that offers to build a model for you. (Of course, all of these elements contributed to why the crisis was ignored for all these years.) Instead, the article recounts a real-life meeting that took place in Goldman Sachs at the end of 2006. The meeting was convened by Goldman CFO, David Viniar, based on some seemingly innocuous happenings. The company had been losing money on its mortgage backed securities for 10 days in a row. The resulting deep-dive into the details of the trades pointed to a sense of unease about the mortgage market. Which then caused Goldman to famously back-off from the market.
I'll leave the reading to you to get more details of what happened. But some thoughts on what contribute to effective risk management practices.
- A real-life feel for the business. You can't be just into the models, you need to be savvy enough to understand how the models you build interact with the real world outside. And it is an appreciation of this interaction that cause the hairs to stand at the back of your neck when you encounter something that just doesn't seem right.
- Proper role of risk management in the decision making hierarchy. Effective risk management takes place when the risk governance has the authority to put the brakes on risk takers (i.e., the traders, in this case). In Goldman, there were a number of enablers for this type of interaction to take place effectively. Most importantly, risk management reported to the CFO, i.e. high enough in the corporate heirarchy. Second, investment decisions needed a go-ahead from both the risk takers and risk governance.
- Mutual respect between risk governance and risk takers. Goldman encourages a collaborative style of decision making. This allows multiple conflicting opinions to be present at the table. Minority opinions are encouraged and appreciated. Over time, this fosters a culture that genuinely tolerates dissonance of opinions. This also allows the CFO to be influenced by the comptroller group as much as he typically would by the trading group.
- Finally, a certain intellectual probity to acknowledge what it does not know or understand. During the meeting, the Goldman team was not able to pinpoint what their source of unease was. But they were able to honestly admit that they didn't really understand what was going on, but that it was also most appropriate to bring the ship to harbour, given their blindspot about what they didn't know. It takes courage to back-off from an investing decision, saying "I don't understand this well enough" in the alpha-male investment banking culture.
All in all, a really interesting read.
Thursday, September 10, 2009
Productivity growth and the never-to-return jobs
I have talked about the economy in a couple of previous posts. This was here talking about green shoots, and about the signs of frailness in the recovery. Over the months of April through August, the life of this blog, news about the economy did seem mixed. The first clear signs that things were beginning to stabilize came around the May timeframe when the drumbeat of negative economic news first started to turn mixed. The jobless claims did not rise as quickly as anticipated, the economy continued to lose jobs but fell off from the rate of close to 0.5 million a month. Around the same time period, existing home sales started to pick up for the first time in more than 2 years and finally in August, the sales pickup translated to rise in prices, for the first time in nearly 2 and a half years.
Meanwhile, Asia continued to power ahead, creating hope and optimism that it would serve as the engine for the stabilization and subsequent growth of the US economy. But even as sectors such as auto, manufacturing and - in some geographies - retail sales have started to show modest increases, job growth still eludes the economy. Quoting the WSJ blog Real Time Economics, a rough sketch of the numbers looks something like this. Average hours worked is declining at an annual rate of nearly 3%, based on quarterly numbers from earlier this year. This is largely driven by the job cuts, but also by anaemic hiring on part of companies. On the other hand, economic indicators point to the GDP growth returning to its cruising rate of about 2-3% a year. The combination of reduced work hours and economic growth translates to a positive growth for this interesting metric called Labor Productivity. One can therefore expect a productivity jump of nearly 4-6% in the third quarter. And given that incomes are flat, this is going to be good news for corporate profits. Dow at 11,000 by the end of year, anyone?
It has been said earlier that this looks like the famous jobless recovery that everyone fears. My take on what is going on. The slumping economy has given corporations the leeway to embrace job automation and computer-driven efficiency measures in a pretty radical manner. The people getting eased out are the ones who have enjoyed a successful run at holding down 'Old Economy' jobs in a world which doesn't value these jobs any longer. When the housing bubble was on, the inefficiency of these jobs never surfaced. But as corporate bottomlines are exposed, companies are making do with fewer and more talented people. Employees who are adept at computers and the use of technology and in its power to ruthlessly driven efficiencies.
For every one of us, this is a sign of how ephemeral our much 'valued' skills are in today's economic reality. A call to action that will be heard by the smart amongst us, but which will also be sadly ignored by many.
Meanwhile, Asia continued to power ahead, creating hope and optimism that it would serve as the engine for the stabilization and subsequent growth of the US economy. But even as sectors such as auto, manufacturing and - in some geographies - retail sales have started to show modest increases, job growth still eludes the economy. Quoting the WSJ blog Real Time Economics, a rough sketch of the numbers looks something like this. Average hours worked is declining at an annual rate of nearly 3%, based on quarterly numbers from earlier this year. This is largely driven by the job cuts, but also by anaemic hiring on part of companies. On the other hand, economic indicators point to the GDP growth returning to its cruising rate of about 2-3% a year. The combination of reduced work hours and economic growth translates to a positive growth for this interesting metric called Labor Productivity. One can therefore expect a productivity jump of nearly 4-6% in the third quarter. And given that incomes are flat, this is going to be good news for corporate profits. Dow at 11,000 by the end of year, anyone?
It has been said earlier that this looks like the famous jobless recovery that everyone fears. My take on what is going on. The slumping economy has given corporations the leeway to embrace job automation and computer-driven efficiency measures in a pretty radical manner. The people getting eased out are the ones who have enjoyed a successful run at holding down 'Old Economy' jobs in a world which doesn't value these jobs any longer. When the housing bubble was on, the inefficiency of these jobs never surfaced. But as corporate bottomlines are exposed, companies are making do with fewer and more talented people. Employees who are adept at computers and the use of technology and in its power to ruthlessly driven efficiencies.
For every one of us, this is a sign of how ephemeral our much 'valued' skills are in today's economic reality. A call to action that will be heard by the smart amongst us, but which will also be sadly ignored by many.
Monday, September 7, 2009
Markets in everything - buying friends
You think you are just too anti-social to cut it in the hypernetworked 21st century? Go and buy your own friends! That's the latest in trying and creating a market for everything.
Read this link. An Australian company will 'sell' you anywhere from a 1000 to 10,000 friends - for a price of course. Creeps me out.
Read this link. An Australian company will 'sell' you anywhere from a 1000 to 10,000 friends - for a price of course. Creeps me out.
Tuesday, September 1, 2009
Knowledge-worker roles in the 21st century - 2/2
I am going to now talk about the second kind of job, that is going to become increasingly attractive for knowledge workers. In the first type of job, I talked about the advances in computing and communication capabilities and technology that make it extremely attractive for jobs that had been performed hitherto by humans to now be transferred to machines. Does this mean that we are all headed into a world depicted in the Matrix or in the Terminator movies?
I think not. As these jobs get outsourced, I anticipate a blowback where society discovers that there are certain types of jobs that cannot be handled by computers at all. These are tasks where highly interrelated decisions need to be made, and where the decisions themselves have second-, third- and fourth-order implications. Also, the situations are such that these implications cannot be 'hard-coded' but keep evolving at a rate that make it necessary for the decision maker to not only follow rules but also exercise judgment. These are places where a 'human touch' is required even in a knowledge role. (I say 'even' because knowledge roles by definition should be easier to codify and outsource to computers.)
One such area that is certainly a judgment based role is risk management. Risk management is anticipating and mitigating different ways in which downside loss can impact a system. Risks can be of two types. One, there are standard 'known' risks whose frequency, pattern of occurence and downside loss impact are comparatively well-known and therefore easier to plan for and mitigate. The second are the unknown risks whose occurency and intensity cannot be predicted. Now any system needs to be set up (if it wants to survive for the long term, that is) to handle both these types of risks. But as you make the system more mechanized to handle the first type of known and predictable risks, it has lesser ability and flexibility to handle the second 'unknown' type of risk.
This is where the role of an experienced risk manager comes in. A risk manager typically has a fair amount of experience in his space. Additionally, he has the ability to maintain mental models of systems in his head which have multiple interactions and whose impacts span multiple time periods. The role of the risk manager is then to devise a system that works equally effectively against both known and unknown risks. The system needs to be such that standard breakdowns are handled without intervention. At the same time, a dashboard of metrics are created about the system which give visibility into the fundamental relationships underlying the system. And when the metrics point to the underlying fundamentals being stretched to breaking point, that's the point at which the occurence of the unexpected risks becomes imminent. The risk manager then steers the system away from being impacted by the downside implications that can result.
My role in my industry is a risk management role, and the role has given me the chance to think deeply about risk and failure modes. And it certainly seems clear to me that there will always be room for human judgment and skills in this domain.
I think not. As these jobs get outsourced, I anticipate a blowback where society discovers that there are certain types of jobs that cannot be handled by computers at all. These are tasks where highly interrelated decisions need to be made, and where the decisions themselves have second-, third- and fourth-order implications. Also, the situations are such that these implications cannot be 'hard-coded' but keep evolving at a rate that make it necessary for the decision maker to not only follow rules but also exercise judgment. These are places where a 'human touch' is required even in a knowledge role. (I say 'even' because knowledge roles by definition should be easier to codify and outsource to computers.)
One such area that is certainly a judgment based role is risk management. Risk management is anticipating and mitigating different ways in which downside loss can impact a system. Risks can be of two types. One, there are standard 'known' risks whose frequency, pattern of occurence and downside loss impact are comparatively well-known and therefore easier to plan for and mitigate. The second are the unknown risks whose occurency and intensity cannot be predicted. Now any system needs to be set up (if it wants to survive for the long term, that is) to handle both these types of risks. But as you make the system more mechanized to handle the first type of known and predictable risks, it has lesser ability and flexibility to handle the second 'unknown' type of risk.
This is where the role of an experienced risk manager comes in. A risk manager typically has a fair amount of experience in his space. Additionally, he has the ability to maintain mental models of systems in his head which have multiple interactions and whose impacts span multiple time periods. The role of the risk manager is then to devise a system that works equally effectively against both known and unknown risks. The system needs to be such that standard breakdowns are handled without intervention. At the same time, a dashboard of metrics are created about the system which give visibility into the fundamental relationships underlying the system. And when the metrics point to the underlying fundamentals being stretched to breaking point, that's the point at which the occurence of the unexpected risks becomes imminent. The risk manager then steers the system away from being impacted by the downside implications that can result.
My role in my industry is a risk management role, and the role has given me the chance to think deeply about risk and failure modes. And it certainly seems clear to me that there will always be room for human judgment and skills in this domain.
Sunday, August 9, 2009
Knowledge-worker roles in the 21st century - 1/2
It is rare to find a piece in the media nowadays that doesn't have a certain "view" on the important social and economic issues of today. Underlying every opinion piece is ideology of some sort. Slavish commitment to the ideology results in the writer typically producing such a biased view that it only appeals to those who are already prejudiced with the same view. It has become extremely difficult (especially with the evolution of the Internet and with blogs) to reach an informed and balanced view on a subject by referencing an authoritative piece on the subject.
Which is why I was pleasantly surprised to come across this piece in the Washington Post today. The piece by Gregory Clark, a professor of economics at UC, Davis presents the view that many of us are afraid to admit. And that is that the US will very soon be forced to confront a reality where the technological advances in the economy today creates its own Haves and Have-Nots. And the chasm between the Haves and Have-Nots would be so huge and so impossible to bridge that the government will be forced to play an equalizing role, so that the social order in society remains more or less intact. So how is new technology creating this chasm? More importantly, for me and my readers, what are the kinds of knowledge-worker jobs that are going to be valued in the twenty-first century?
The last fifty years of the second millenium have been marked by the emergence of the computer. A machine designed to do millions of logical and mathematical operations in a fraction of a second, the computer has now started to take over a vast majority of the computing and logical thinking that human beings would usually perform. With the ability of the computer (through programming languages) to execute long sequences of operations at high speed, the end-result is a powerful "proxy" intelligence that can be harnessed to do both good and harm. And this proxy-intelligence is taking the place of traditional intelligence; the role performed by human beings in society. And this intelligence comes without moods, expectations of recognition/ praise; in fact, without any kind of the emotional inconsistencies and quirks shown by human beings. No surprise that many of the front-end business processes involving the delivery of basic and transactional services to consumers is being replaced by the computer (such as the ATM machine). With the computer becoming an increasingly integral part of the economy, I see two kinds of jobs that knowledge-workers can embrace in this economy. I am going to cover one of these roles in this post and the second, in the next post.
The first role is that of the accelarator towards an increasing automation of simple business processes. The cost benefit of the computer over human beings is obvious; however in order for the computer to perform even in a limited way like human beings, detailed instruction sets with logical end-points at each node need to be created. It requires the imagination and creativity of the human mind to do this programming in a really effective manner - i.e. the computer actually being able to do what the human being in the same position would have been able to do. Also, it requires human ingenuity to engineer the machine to do this efficiently - within the desired speed and operating cost constraints. This role of an accelarator or an enabler of the "outsourcing" of hitherto human performed activity to machines will be increasingly in demand over the next 10-15 years.
This role will require a unique mix of skills. First and foremost, the role requires a detailed understanding of business processes, the roles played by the various players, the inputs and outputs at various stages. The business process understanding needs to span multiple companies and industries. Let's take something that Clark refers in his article: change to a flight reservation. The business process calls for not just access to the reservations database and the flights database, but also things like changing meal options, providing seating information (with information about the aircraft seating chart), reconfirming the frequent flyer account number, etc. Additionally, providing options for payment if there is going to be a fee involved.
Second, the role requires the ability to understand the capabilities of IT platforms and packages to able to perform the desired function. This role actually has two components. One is the mapping of human actions into the logic understood by a computer system. The second is the system architecture/ engineering side, which is the configuration of the various building blocks (comprised of different IT "boxes" delivering different functionality) to create an end-to-end process delivery capability. Given the lack of standards that exist for these types of solutions, any deep skills in this area involves understanding the peculiarities of specific solutions in a great level of detail.
I'd love to hear more from readers on this. Have you seen these roles emerging in your industry? What other types of skills does the enabler or accelarator role need?
Which is why I was pleasantly surprised to come across this piece in the Washington Post today. The piece by Gregory Clark, a professor of economics at UC, Davis presents the view that many of us are afraid to admit. And that is that the US will very soon be forced to confront a reality where the technological advances in the economy today creates its own Haves and Have-Nots. And the chasm between the Haves and Have-Nots would be so huge and so impossible to bridge that the government will be forced to play an equalizing role, so that the social order in society remains more or less intact. So how is new technology creating this chasm? More importantly, for me and my readers, what are the kinds of knowledge-worker jobs that are going to be valued in the twenty-first century?
The last fifty years of the second millenium have been marked by the emergence of the computer. A machine designed to do millions of logical and mathematical operations in a fraction of a second, the computer has now started to take over a vast majority of the computing and logical thinking that human beings would usually perform. With the ability of the computer (through programming languages) to execute long sequences of operations at high speed, the end-result is a powerful "proxy" intelligence that can be harnessed to do both good and harm. And this proxy-intelligence is taking the place of traditional intelligence; the role performed by human beings in society. And this intelligence comes without moods, expectations of recognition/ praise; in fact, without any kind of the emotional inconsistencies and quirks shown by human beings. No surprise that many of the front-end business processes involving the delivery of basic and transactional services to consumers is being replaced by the computer (such as the ATM machine). With the computer becoming an increasingly integral part of the economy, I see two kinds of jobs that knowledge-workers can embrace in this economy. I am going to cover one of these roles in this post and the second, in the next post.
The first role is that of the accelarator towards an increasing automation of simple business processes. The cost benefit of the computer over human beings is obvious; however in order for the computer to perform even in a limited way like human beings, detailed instruction sets with logical end-points at each node need to be created. It requires the imagination and creativity of the human mind to do this programming in a really effective manner - i.e. the computer actually being able to do what the human being in the same position would have been able to do. Also, it requires human ingenuity to engineer the machine to do this efficiently - within the desired speed and operating cost constraints. This role of an accelarator or an enabler of the "outsourcing" of hitherto human performed activity to machines will be increasingly in demand over the next 10-15 years.
This role will require a unique mix of skills. First and foremost, the role requires a detailed understanding of business processes, the roles played by the various players, the inputs and outputs at various stages. The business process understanding needs to span multiple companies and industries. Let's take something that Clark refers in his article: change to a flight reservation. The business process calls for not just access to the reservations database and the flights database, but also things like changing meal options, providing seating information (with information about the aircraft seating chart), reconfirming the frequent flyer account number, etc. Additionally, providing options for payment if there is going to be a fee involved.
Second, the role requires the ability to understand the capabilities of IT platforms and packages to able to perform the desired function. This role actually has two components. One is the mapping of human actions into the logic understood by a computer system. The second is the system architecture/ engineering side, which is the configuration of the various building blocks (comprised of different IT "boxes" delivering different functionality) to create an end-to-end process delivery capability. Given the lack of standards that exist for these types of solutions, any deep skills in this area involves understanding the peculiarities of specific solutions in a great level of detail.
I'd love to hear more from readers on this. Have you seen these roles emerging in your industry? What other types of skills does the enabler or accelarator role need?
Monday, August 3, 2009
Why individual level data analysis is difficult
I recently completed a piece of data analysis using individual level data. The project was one of the more challenging pieces of analysis I have undertaken at work, and I was (happily, for myself and everyone else who worked on it) able to turn it into something useful. And there were some good lessons learned at the end of it all, which I want to share in today's post.
So, what is unique and interesting about individual level data and why is it valuable?
- With any dataset that you want to derive insights from, there are a number of attributes about the unit being analyzed (individual, group, state, country) and one or more attributes that you are trying to explain. Let's call the first group predictors and the second group target variables. Individual level data has a much wider range of predictor and target variables. There is also a much wider range of interactions between these various predictors. For example, while on an average, older people tend to be wealthier, individual level data reveals that there are older people who are broke and younger people who are millionaires. As a result of these wide ranges of data and the different types of interactions between these variables (H-L, M-M, M-H, L-H ... you get the picture), it is possible to understand fundamental relationships between the predictors and the targets and interactions between the predictors. Digging a little deeper into the people vs wealth data, what this might tell you is that what really matters for your level of wealth is your education levels, the type of job you do, etc. This level of variation is not available with the group level data. In other words, the group level data is just not as rich.
- Now, along with the upside comes downside. The richness of the individual level predictors means that data occassionally is messy. What is messy? Messy means having wrong values at an individual level, sometimes missing or null values at an individual level. At a group level, many of the mistakes average themselves out, especially if the errors are distributed evenly around zero. But at the individual levels, the likelihood of errors has to be managed as part of the analysis work. With missing data, the challenge is magnified. Is missing data truly missing? Or did it get dropped during some data gathering step? Is there something systematic to missing data, or is it random? Should missing data be treated as missing or should it be imputed to some average value? Or should it be imputed to a most likely value? These are all things that can materially impact the analysis and therefore should be given due consideration.
Now to the analysis itself. What were some of my important lessons?
- Problem formulation has to be crystal clear and that in turn should drive the technique.
Problem formulation is the most important step of the problem solving exercise. What are we trying to do with the data? Are we trying to build a predictive model with all the data? Are we examining interactions between predictors? Are we studying the relationship between predictors one at a time and the target? All of these outcomes require different analytical approaches. Sometimes, analysts learn a technique and then look around for a nail to hit. But judgment is needed to make sure the appropriate technique is used. The analyst needs to have the desire to learn to use an technique that he/she is not aware of. By the same token, discipline to use a simpler technique where appropriate.
- Spending time to understand the data is a big investment that is completely worth it.
You cannot spend too much time understanding the data. Let me repeat that for effect. You cannot spend too much time understanding the data. And I have come to realize that far from being a drudge, understanding the data is one of the most fulfilling and value added pieces of any type of analysis. The most interesting part of understanding data (for me) is the sheer number of data points that are located so far away from the mean or median of the sample. So if you are looking at people with mortgages and the average mortgage amount is $150,000, the number of cases where the mortgage amount exceeds $1,000,000 lends a completely new perspective of the type of people in your sample.
- Explaining the results in a well-rounded manner is a critical close-out at the end.
The result of a statistical analysis is usually a set of predictors which have met the criteria for significance. Or it could be a simple two variable correlation that is above a certain threshold. But whatever be the results of the analysis, it is important to base the analysis result in real-life insights that can be understood by the audience. So, if the insight reveals that people with large mortgages have a higher propensity to pay off their loans, further clarification will be useful around the income level of these people, their education levels, the types of jobs they hold, etc. All these ancillary data points are ways of closing out the profile of the "thing" that has been revealed by the analysis.
- Speed is critical to get the results in front of the right people before they lose interest.
And finally, if you are going to spend a lot of everyone's (and your) precious time doing a lot of the above, the results need to be driven in extra-short time for people to keep their interest in what you are doing. In today's information-saturated world, it only takes the next headline in the WSJ for people to start talking about something else. So, you need to basically do the analysis in a smart manner, and also it needs to be super-fast. Delivered yesterday, as the cliche goes.
In hindsight, it gives me an appreciation of why data analysis or statistical analysis using individual level data is one of the more challenging analytical exercises. And why it is so difficult to get it right.
So, what is unique and interesting about individual level data and why is it valuable?
- With any dataset that you want to derive insights from, there are a number of attributes about the unit being analyzed (individual, group, state, country) and one or more attributes that you are trying to explain. Let's call the first group predictors and the second group target variables. Individual level data has a much wider range of predictor and target variables. There is also a much wider range of interactions between these various predictors. For example, while on an average, older people tend to be wealthier, individual level data reveals that there are older people who are broke and younger people who are millionaires. As a result of these wide ranges of data and the different types of interactions between these variables (H-L, M-M, M-H, L-H ... you get the picture), it is possible to understand fundamental relationships between the predictors and the targets and interactions between the predictors. Digging a little deeper into the people vs wealth data, what this might tell you is that what really matters for your level of wealth is your education levels, the type of job you do, etc. This level of variation is not available with the group level data. In other words, the group level data is just not as rich.
- Now, along with the upside comes downside. The richness of the individual level predictors means that data occassionally is messy. What is messy? Messy means having wrong values at an individual level, sometimes missing or null values at an individual level. At a group level, many of the mistakes average themselves out, especially if the errors are distributed evenly around zero. But at the individual levels, the likelihood of errors has to be managed as part of the analysis work. With missing data, the challenge is magnified. Is missing data truly missing? Or did it get dropped during some data gathering step? Is there something systematic to missing data, or is it random? Should missing data be treated as missing or should it be imputed to some average value? Or should it be imputed to a most likely value? These are all things that can materially impact the analysis and therefore should be given due consideration.
Now to the analysis itself. What were some of my important lessons?
- Problem formulation has to be crystal clear and that in turn should drive the technique.
Problem formulation is the most important step of the problem solving exercise. What are we trying to do with the data? Are we trying to build a predictive model with all the data? Are we examining interactions between predictors? Are we studying the relationship between predictors one at a time and the target? All of these outcomes require different analytical approaches. Sometimes, analysts learn a technique and then look around for a nail to hit. But judgment is needed to make sure the appropriate technique is used. The analyst needs to have the desire to learn to use an technique that he/she is not aware of. By the same token, discipline to use a simpler technique where appropriate.
- Spending time to understand the data is a big investment that is completely worth it.
You cannot spend too much time understanding the data. Let me repeat that for effect. You cannot spend too much time understanding the data. And I have come to realize that far from being a drudge, understanding the data is one of the most fulfilling and value added pieces of any type of analysis. The most interesting part of understanding data (for me) is the sheer number of data points that are located so far away from the mean or median of the sample. So if you are looking at people with mortgages and the average mortgage amount is $150,000, the number of cases where the mortgage amount exceeds $1,000,000 lends a completely new perspective of the type of people in your sample.
- Explaining the results in a well-rounded manner is a critical close-out at the end.
The result of a statistical analysis is usually a set of predictors which have met the criteria for significance. Or it could be a simple two variable correlation that is above a certain threshold. But whatever be the results of the analysis, it is important to base the analysis result in real-life insights that can be understood by the audience. So, if the insight reveals that people with large mortgages have a higher propensity to pay off their loans, further clarification will be useful around the income level of these people, their education levels, the types of jobs they hold, etc. All these ancillary data points are ways of closing out the profile of the "thing" that has been revealed by the analysis.
- Speed is critical to get the results in front of the right people before they lose interest.
And finally, if you are going to spend a lot of everyone's (and your) precious time doing a lot of the above, the results need to be driven in extra-short time for people to keep their interest in what you are doing. In today's information-saturated world, it only takes the next headline in the WSJ for people to start talking about something else. So, you need to basically do the analysis in a smart manner, and also it needs to be super-fast. Delivered yesterday, as the cliche goes.
In hindsight, it gives me an appreciation of why data analysis or statistical analysis using individual level data is one of the more challenging analytical exercises. And why it is so difficult to get it right.
Tuesday, July 21, 2009
More data visualization - this time about books
Ever wonder where the proof was about reading ..ummm, erotica being bad for you. Here it is. Check this link out.
An interesting study was done that went somewhat like this.
- Get the ten most frequent "favorite books" at every college using the college's Network Statistics page on Facebook. Possibly these books represent the intellectual calibre of the college.
- Get their SAT/ACT scores for the colleges.
- You can now get a relationship between types of book read and scholastic achievement
The results are pretty impressive, though still somewhat dubious. According to the study, Classics is usually good for you (agree with that), Erotica is way bad. Controversially, so is African-American literature and chick-lit. In the link, check out the visual that stacks the book by genre.
Make what you want about this, but be careful between causality and correlation.
An interesting study was done that went somewhat like this.
- Get the ten most frequent "favorite books" at every college using the college's Network Statistics page on Facebook. Possibly these books represent the intellectual calibre of the college.
- Get their SAT/ACT scores for the colleges.
- You can now get a relationship between types of book read and scholastic achievement
The results are pretty impressive, though still somewhat dubious. According to the study, Classics is usually good for you (agree with that), Erotica is way bad. Controversially, so is African-American literature and chick-lit. In the link, check out the visual that stacks the book by genre.
Make what you want about this, but be careful between causality and correlation.
Saturday, July 18, 2009
Data visualization
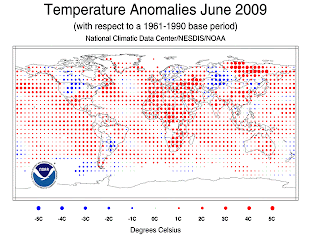
An example of a really well-done graphic is from the NOAA website. Science and particularly math afficionados seem to have a particular affinity to following weather science. (I am wondering whether it is a visceral reaction to global warming naysayers who, the scientists think, are possibly insulting their learning.)
The graph is a world temperature graph and this type of graph has come in so many different forms, it is difficult not to have seen such a graph. What I like about this is the elegant and non-intrusive form in which the overlays are done.
• By using dots and varying the size of the dots, the creator of the graph is making sure that the underlying geographic details (important in a world map where there is great detail that needs to be captured in a small area, therefore you cannot use very thick lines for country borders) still come through.
• The other thing that I liked is some of the simplications the creator has made. The dots are equally spaced but I am pretty sure that’s exactly not how the data was gathered. But to tell the story, that detail is not as important.
The graphic came from Jeff Masters' weather blog which is one of the best of its kind. Here's a link if you are interested.
Wednesday, July 15, 2009
Two great finds for physics fans
Back after a long break in the posts. Call it a mixture of home responsibilities, writer's block and just some plain old laziness.
One of my other interests (apart from statistics and social science) is physics and technology. I really enjoy reading about emerging applications of technology in various spheres of social and economic importance. The Technology Quarterly of the Economist is one of my treasured reads (though I end up reading very little of it, because of me wanting to leave aside "quality time" to do the reading).
I want to share two recent finds in the science and physics space. One is a really good book called "The Great Equations" by Robert Crease. The book covers ten of the seminal equations in physics and basically spins a story around how the equation formulator came about to creating the equation. There is usually a little mathematical proof behind the story usually, but most of the book is about the professional journey made by the scientist from an existing view of the world (or an older paradigm, to be more exact) to a new paradigm. And the paradigm is usually encapsulated in the form of an equation.
I found a couple of aspects about the journey extremely interesting. One, it was fascinating to have a window into the minds of physics greats (Newton, Maxwell, Einstein, Schrodinger, to name a few) and see how they synthesized the various different world views around them to create or arrive at their respective equations. The ability to deal with all the complexity of observed phenomena, the different philosophies and world views and to come up with something as elegant as a great equation, that defines genius for me. The second aspect that I found extremely interesting was that there was usually years and years of experimentation or mathematical work that preceded arriving at the great equation. One might be inclined to think that the great equations (given their utter simplicity) happen through a flash of inspiration. Nothing could be further from the truth.
The next find were the Feynman lectures. Now, many of us have read some of the Feynman lectures or have seen the lectures on a place like Youtube. But how cool would it be to have these lectures be annotated by Bill Gates? Check this link out at the Microsoft Research website. And happy watching!
I am guessing this blog has a fair share of aspiring or one-time physics and engineering fans. How do you keep your engineering bone tickled? I'd love to hear your pet indulgences.
One of my other interests (apart from statistics and social science) is physics and technology. I really enjoy reading about emerging applications of technology in various spheres of social and economic importance. The Technology Quarterly of the Economist is one of my treasured reads (though I end up reading very little of it, because of me wanting to leave aside "quality time" to do the reading).
I want to share two recent finds in the science and physics space. One is a really good book called "The Great Equations" by Robert Crease. The book covers ten of the seminal equations in physics and basically spins a story around how the equation formulator came about to creating the equation. There is usually a little mathematical proof behind the story usually, but most of the book is about the professional journey made by the scientist from an existing view of the world (or an older paradigm, to be more exact) to a new paradigm. And the paradigm is usually encapsulated in the form of an equation.
I found a couple of aspects about the journey extremely interesting. One, it was fascinating to have a window into the minds of physics greats (Newton, Maxwell, Einstein, Schrodinger, to name a few) and see how they synthesized the various different world views around them to create or arrive at their respective equations. The ability to deal with all the complexity of observed phenomena, the different philosophies and world views and to come up with something as elegant as a great equation, that defines genius for me. The second aspect that I found extremely interesting was that there was usually years and years of experimentation or mathematical work that preceded arriving at the great equation. One might be inclined to think that the great equations (given their utter simplicity) happen through a flash of inspiration. Nothing could be further from the truth.
The next find were the Feynman lectures. Now, many of us have read some of the Feynman lectures or have seen the lectures on a place like Youtube. But how cool would it be to have these lectures be annotated by Bill Gates? Check this link out at the Microsoft Research website. And happy watching!
I am guessing this blog has a fair share of aspiring or one-time physics and engineering fans. How do you keep your engineering bone tickled? I'd love to hear your pet indulgences.
Wednesday, July 8, 2009
Market chills
I have argued in a number of recent posts: here, here and here that we are nowhere close to the bottom when it comes to this economic downturn. The jobless numbers are back to sliding downwards at an accelerated pace after one month of deceleration.
And the markets seem to have caught the chills.
We discussed this at work a few months back. Someone who is very well-respected in banking circles and who has seen a few past recessions called out that you can tell that a recovery is underway when there is a sustained period where the indicators yo-yo between good and bad news. We seem to be entering this phase now.
And the markets seem to have caught the chills.

We discussed this at work a few months back. Someone who is very well-respected in banking circles and who has seen a few past recessions called out that you can tell that a recovery is underway when there is a sustained period where the indicators yo-yo between good and bad news. We seem to be entering this phase now.
Friday, July 3, 2009
Best Coffee Survey and a research methodology question
A recent Zagat survey rated the best coffee in the US. The best coffee rating went (expectedly, I guess) to Starbucks. Even though I have had better coffee at other places, I guess Starbucks combines great coffee with ubiquitous presence and therefore ends up getting the top rating. Now, I think Starbucks coffee is good and the baristas are extremely friendly, but in terms of pure coffee flavour, I would rate Panera's Hazelnut coffee higher. Also some of the Kona coffees that you find at places like WaWa are also really good. Any kind of place serving Jamaica's Blue Mountain coffee will obviously be great. So what makes Starbucks special? Are there other factors at play beyond the pure taste of the coffee.
One hypothesis is that the national-level presence of Starbucks could be contributing towards the voting going for Starbucks. In places where Starbucks has to compete with other chains like Peets (San Francisco) and Dunkin Donuts (New England), comparative ratings between Starbucks and other chains shows a narrower gap. In places where Starbucks has not competition however, it is likely to get disproportionately good ratings.
Let us say you are one of the contributors in the survey and are in St.Louis, MO. The competition for Starbucks in St.Louis is likely to be (I guess) the burnt robusta coffee at the local restaurant. In such a market, Starbucks will enjoy a clear advantage, both for the quality of the coffee as well as the ambience. So, let's say, you had to rate Starbucks on a scale of 1-5. It is likely you would give Starbucks a 4-5 in a non-competitive market, such as St.Louis, in the absence of valid benchmarks or competition to compare against. In a competitive market dominated by multiple brands, the difference between Starbucks and other brands is likely to be narrower. Also, the assertion can also be made that a more discerning audience (having had the opportunity to sample multiple chains) is less likely to give extremely high scores (4s and 5s) to any of the choices under consideration.
Therefore, the sampling design and the analysis methodology becomes extremely critical for surveys around this. To avoid the "no-competition" bias, there could a number of questions a market research analyst would need to ask herself:
1. Should we use only data points from places where there are multiple chains in the same geography? (Doesn't sound fair. We will be throwing away data, which a lot of sensible people have explained is a cardinal sin. We should probably weight the information in some way).
2. Should we consider data for the analysis only where a person has provided ratings about multiple chains voluntarily or penalize when people have not rated a chain that could have been rated?
3. Or are there modeling solutions available to manage this conundrum? Topic of my next post!
One hypothesis is that the national-level presence of Starbucks could be contributing towards the voting going for Starbucks. In places where Starbucks has to compete with other chains like Peets (San Francisco) and Dunkin Donuts (New England), comparative ratings between Starbucks and other chains shows a narrower gap. In places where Starbucks has not competition however, it is likely to get disproportionately good ratings.
Let us say you are one of the contributors in the survey and are in St.Louis, MO. The competition for Starbucks in St.Louis is likely to be (I guess) the burnt robusta coffee at the local restaurant. In such a market, Starbucks will enjoy a clear advantage, both for the quality of the coffee as well as the ambience. So, let's say, you had to rate Starbucks on a scale of 1-5. It is likely you would give Starbucks a 4-5 in a non-competitive market, such as St.Louis, in the absence of valid benchmarks or competition to compare against. In a competitive market dominated by multiple brands, the difference between Starbucks and other brands is likely to be narrower. Also, the assertion can also be made that a more discerning audience (having had the opportunity to sample multiple chains) is less likely to give extremely high scores (4s and 5s) to any of the choices under consideration.
Therefore, the sampling design and the analysis methodology becomes extremely critical for surveys around this. To avoid the "no-competition" bias, there could a number of questions a market research analyst would need to ask herself:
1. Should we use only data points from places where there are multiple chains in the same geography? (Doesn't sound fair. We will be throwing away data, which a lot of sensible people have explained is a cardinal sin. We should probably weight the information in some way).
2. Should we consider data for the analysis only where a person has provided ratings about multiple chains voluntarily or penalize when people have not rated a chain that could have been rated?
3. Or are there modeling solutions available to manage this conundrum? Topic of my next post!
Sunday, June 28, 2009
Research funding or Why we have still haven't found a cure for cancer
Cancer has ben known to medicine since the time of Hippocrates. And modern medicine has known and studies causes of cancer since the mid-18th century. In 1971, Nixon announced a project to create a cure for cancer and (a la Kennedy, with regard to the moon mission) announced a definite cure in the next five years. Today, nearly 40 years and $105 billion dollars of public investment later (the private investment can be considered to be at least a significant fraction the public investment), we are no closer to finding a cure. In fact, after adjusting for age and size of the population, the cancer death rate has dropped by only 5% in the last 50 years. Compare this with nearly 60% drop in the death rates of diseases like pneumonia and influenza. Why is this the case?
Part of the reason is that cancer has multiple causes and we are not really sure about the true causal linkage between the various factors and the cancer cells misbehaving. Environmental factors cause some types, exposure to radioactivity causes other types, tobacco is a well-known factor causing mouth and lung cancer and there are viruses that cause still some other types. The common thread linking all of these causal factors and the various different types of cancers they cause is difficult to isolate. And therefore while we continue to make some improvements around the margin (getting people to live for a few additional months or years), a true cure has been elusive.
But another likely cause is the way in which various research funding agencies have made investment prioritization decisions. The funds have invariably gone to small-budget, incremental improvement type projects which are usually along previously established avenues of inquiry. The truly innovative approaches and especially the risky (from a success of the project standpoint) proposals have seldom obtained funding. The process developed to identify research subjects have been good at avoiding funding truly bad research. However, by the same token, they have continued to fund projects that are conventional and low risk and as a result, only contributing to marginal improvements. A recent article in the New York Times sheds some more light on to this topic.
My view is that this is quite a common problem (sub-optimal funds allocation) when funds are limited. This is not only true for cancer research in particular or any other form of medical research in general, but even in the financial services industry that I am part of. The funds allocation agency feels pressure not to waste the limited funds and also to make sure that the maximum amount of research projects get the benefits of the fund. Therefore the push to fund projects that are from proven areas and are set up to make incremental improvements to the areas. Also, this leads to a tendency to parcel the funds and distribute small quantities into a large number of projects, While what they should be paradoxically doing (given the shortage of funds) is to make the bold bet and fund those areas which may not be as proven but show the highest promise for overall success. Again, this happens more commonly than in the field of cancer research.
Challenging the financial budget, the status-quo way of thinking is not easy to do. There will be people who will say No and be discouraging, rarely because them have something to lose but mostly because the tendency is to play safe. People usually do not get fired for taking the safe, conventional-wisdom driven decisions. It is the risk-takers that get panned if the risks do not play out as expected.
Part of the reason is that cancer has multiple causes and we are not really sure about the true causal linkage between the various factors and the cancer cells misbehaving. Environmental factors cause some types, exposure to radioactivity causes other types, tobacco is a well-known factor causing mouth and lung cancer and there are viruses that cause still some other types. The common thread linking all of these causal factors and the various different types of cancers they cause is difficult to isolate. And therefore while we continue to make some improvements around the margin (getting people to live for a few additional months or years), a true cure has been elusive.
But another likely cause is the way in which various research funding agencies have made investment prioritization decisions. The funds have invariably gone to small-budget, incremental improvement type projects which are usually along previously established avenues of inquiry. The truly innovative approaches and especially the risky (from a success of the project standpoint) proposals have seldom obtained funding. The process developed to identify research subjects have been good at avoiding funding truly bad research. However, by the same token, they have continued to fund projects that are conventional and low risk and as a result, only contributing to marginal improvements. A recent article in the New York Times sheds some more light on to this topic.
My view is that this is quite a common problem (sub-optimal funds allocation) when funds are limited. This is not only true for cancer research in particular or any other form of medical research in general, but even in the financial services industry that I am part of. The funds allocation agency feels pressure not to waste the limited funds and also to make sure that the maximum amount of research projects get the benefits of the fund. Therefore the push to fund projects that are from proven areas and are set up to make incremental improvements to the areas. Also, this leads to a tendency to parcel the funds and distribute small quantities into a large number of projects, While what they should be paradoxically doing (given the shortage of funds) is to make the bold bet and fund those areas which may not be as proven but show the highest promise for overall success. Again, this happens more commonly than in the field of cancer research.
Challenging the financial budget, the status-quo way of thinking is not easy to do. There will be people who will say No and be discouraging, rarely because them have something to lose but mostly because the tendency is to play safe. People usually do not get fired for taking the safe, conventional-wisdom driven decisions. It is the risk-takers that get panned if the risks do not play out as expected.
Thursday, June 25, 2009
Why I blog
I have been regular at maintaining the blog for the past month or so. My feelings have been mixed so far. On the one hand, it is an effort to keep up the writing effort day after day. One of my goals is to make sure that the blog remains fresh for future readers. And the freshness of the blog is to totally a function of keeping up the effort of adding new and interesting material. With my day job and with the challenges of keeping up with the ever growing demands of our 5-month old, writing is always not easy.
But being a glass-half-full kind of guy, what has this exercise brought me?
For starters, it has got me to start writing again. I am a firm believer that writing is a great way of organizing your thoughts and making them more logical and structured. And it is a habit that I had at one time, lost at some point and am keen on regaining again. Communication is an important skill in today's world. With all the clutter, media generated noise, terabytes of data and messages flowing back and forth, the Internet driven distractions, it is important to cut through the clutter and reach out powerfully with one's words to make a difference. Gandhi had a difficult enough time getting his word out to millions of his countrymen and getting them united against the British. But that was close to a century back. Imagine Obama's difficulty in getting his thoughts out to people in today's hyper-information age. And the way you get better at communication is by keeping at it through weekdays and weekends, through work deadlines and daughter's shrieks of excitement. Hopefully, I am getting better at this stuff.
The other big positive for me is that I am beginning to learn a lot more and at a much faster pace on my professional interest, math models and statistical inference. As Hal Varian, chief economist at Google has famously remarked, the statistician job is going to be the sexy job for the next ten years. And this field is evolving so rapidly that it is extremely critical to keep updating one's knowledge and skills and remaining ahead of the curve. In order for me to provide a stream of meaningful material for the audience of this blog, I have had to spend a good amount of my time reading and updating my own knowledge base. Just last morning, I managed to read an interesting article on multi-level modeling. This took me to a web-site dedicated to multi-level modeling at the University of Bristol. And the lecture notes in turn made me aware of some of the ways I could tackle some ticklish problems at work. (Look at this really cool lecture. It is a video link and needs Internet Explorer as the browser.) I have become much more aware of the latest problems and solution kits out there in the last month, than what I learnt in the past several years. A huge plus for me.
So all-in-all, I am hoping to learn something out of all this and make at least a fractional improvement to what I want to add to the world. And hopefully keep my audience engaged and interested in the stuff I write. My writings are clearly not meant for the masses, I don't have any such hopes! The people who are likely to like my writing are going to be similar to me: numbers-obsessed, math-loving and tech savvy geeks. And if I can make a fraction of a difference to my readers as this exercise is making for me, I will be a happy blogger.
But being a glass-half-full kind of guy, what has this exercise brought me?
For starters, it has got me to start writing again. I am a firm believer that writing is a great way of organizing your thoughts and making them more logical and structured. And it is a habit that I had at one time, lost at some point and am keen on regaining again. Communication is an important skill in today's world. With all the clutter, media generated noise, terabytes of data and messages flowing back and forth, the Internet driven distractions, it is important to cut through the clutter and reach out powerfully with one's words to make a difference. Gandhi had a difficult enough time getting his word out to millions of his countrymen and getting them united against the British. But that was close to a century back. Imagine Obama's difficulty in getting his thoughts out to people in today's hyper-information age. And the way you get better at communication is by keeping at it through weekdays and weekends, through work deadlines and daughter's shrieks of excitement. Hopefully, I am getting better at this stuff.
The other big positive for me is that I am beginning to learn a lot more and at a much faster pace on my professional interest, math models and statistical inference. As Hal Varian, chief economist at Google has famously remarked, the statistician job is going to be the sexy job for the next ten years. And this field is evolving so rapidly that it is extremely critical to keep updating one's knowledge and skills and remaining ahead of the curve. In order for me to provide a stream of meaningful material for the audience of this blog, I have had to spend a good amount of my time reading and updating my own knowledge base. Just last morning, I managed to read an interesting article on multi-level modeling. This took me to a web-site dedicated to multi-level modeling at the University of Bristol. And the lecture notes in turn made me aware of some of the ways I could tackle some ticklish problems at work. (Look at this really cool lecture. It is a video link and needs Internet Explorer as the browser.) I have become much more aware of the latest problems and solution kits out there in the last month, than what I learnt in the past several years. A huge plus for me.
So all-in-all, I am hoping to learn something out of all this and make at least a fractional improvement to what I want to add to the world. And hopefully keep my audience engaged and interested in the stuff I write. My writings are clearly not meant for the masses, I don't have any such hopes! The people who are likely to like my writing are going to be similar to me: numbers-obsessed, math-loving and tech savvy geeks. And if I can make a fraction of a difference to my readers as this exercise is making for me, I will be a happy blogger.
Monday, June 22, 2009
... the Great Escape? Or the Great Deception?
In an earlier post, I commented on the now famous "Green Shoots" of recovery but the very real long term threats to continued economic growth. It turns out that the "so-called" economic recovery seems to be more of a financial market recovery. Conventional wisdom goes that the financial markets turnaround precedes the real economy turnaround by about 6 months. Early signs did point to this phenomenon. Market indices in both emerging markets and the developed markets showed smart 30%+ growths in the last 3 months. Corporate bond offerings began to surge and even below investment grade offerings jumped up (and were well subscribed) in June.
However, some temperance seem to have set in of late. Emerging market indices like the Sensex and the Hang Seng are at least about 10-15% down from their early June peaks. Likewise with the DJIA. The steady upper trend seen for the best part of the last 8 weeks seems to have been interrupted. The yield on 10-year US treasuries had gone up to nearly 4% but is not trended back down to about 3.5%, basically signalling that everything is not as hunky-dory as we expected. There is still a high demand for quality (the irony of it all is that quality is denoted by US treasuries!). The Economist states that all economic indicators have not magically turned to positive, which is what one would expect if the markets and the media are to be believed. According to the Economist,
The June Empire State survey of manufacturing activity in New York showed a retreat. German export figures for April showed a 4.8% month-on-month fall. The latest figures for American and euro-zone industrial production showed similar dips. American raw domestic steel production is down 47% year on year; railway traffic in May was almost a quarter below its level of a year earlier. Bankers say that chief executives seem a lot less confident about the existence of “green shoots” than markets are.
We shouldn't be either. For a bunch of reasons.
1. Losses are nowhere close to bottoming out. Expectations for large credit defaults amongst corporates is expected to be higher than 11% for 2009 and continue to remain there for 2010.
2. At the individual level, unemployment is showing no signs of abating. There was a good article in the Washington Post today on how the economic recovery seems to be taking place in the absence of jobs. Check this link out. Unemployment is expected to be north of 10% and remain there for a good part of 2009 and into 2010. Unemployment is closely linked with the consumer confidence number and therefore any sluggishness in the job market is going to impact consumer spending and therefore further impact the rate of recovery of the economy.
3. Emerging markets were the promised land for the world economy, not not any longer. The markets don't seem to think so however. Indian economic growth is expected to be the slowest in the past 6 years. With much more fragile safety nets in the Asian economic tigers, these economies are going to be even more careful while navigating out of the downturn.
In short, a long haul seems clear. Also seems clear is a fundamental remaking of industries as a whole. Financial services, automobiles and potentially health-care are industries where a new business model is ripe for discovery. This should create many more opportunities for the data scientist, the topic of my next post.
However, some temperance seem to have set in of late. Emerging market indices like the Sensex and the Hang Seng are at least about 10-15% down from their early June peaks. Likewise with the DJIA. The steady upper trend seen for the best part of the last 8 weeks seems to have been interrupted. The yield on 10-year US treasuries had gone up to nearly 4% but is not trended back down to about 3.5%, basically signalling that everything is not as hunky-dory as we expected. There is still a high demand for quality (the irony of it all is that quality is denoted by US treasuries!). The Economist states that all economic indicators have not magically turned to positive, which is what one would expect if the markets and the media are to be believed. According to the Economist,
The June Empire State survey of manufacturing activity in New York showed a retreat. German export figures for April showed a 4.8% month-on-month fall. The latest figures for American and euro-zone industrial production showed similar dips. American raw domestic steel production is down 47% year on year; railway traffic in May was almost a quarter below its level of a year earlier. Bankers say that chief executives seem a lot less confident about the existence of “green shoots” than markets are.
We shouldn't be either. For a bunch of reasons.
1. Losses are nowhere close to bottoming out. Expectations for large credit defaults amongst corporates is expected to be higher than 11% for 2009 and continue to remain there for 2010.
2. At the individual level, unemployment is showing no signs of abating. There was a good article in the Washington Post today on how the economic recovery seems to be taking place in the absence of jobs. Check this link out. Unemployment is expected to be north of 10% and remain there for a good part of 2009 and into 2010. Unemployment is closely linked with the consumer confidence number and therefore any sluggishness in the job market is going to impact consumer spending and therefore further impact the rate of recovery of the economy.
3. Emerging markets were the promised land for the world economy, not not any longer. The markets don't seem to think so however. Indian economic growth is expected to be the slowest in the past 6 years. With much more fragile safety nets in the Asian economic tigers, these economies are going to be even more careful while navigating out of the downturn.
In short, a long haul seems clear. Also seems clear is a fundamental remaking of industries as a whole. Financial services, automobiles and potentially health-care are industries where a new business model is ripe for discovery. This should create many more opportunities for the data scientist, the topic of my next post.
Saturday, June 20, 2009
Monte Carlo simulations gone bad
In my series on stress testing models, I concluded with Monte Carlo simulations as a way of understanding the set of outcomes a model can produce and being able to handle a wide set of inputs without breaking down. However, Monte Carlo simulations can be done in ways that at best, are totally useless and at worst, can produce highly misleading outcomes. I want to discuss some of these breakdown modes in this post.
So, (drumroll), top Monte Carlo simulation fallacies I have come across.
1. Assuming all of the model drivers are normally distributed
Usually the biggest fallacy of them all. I have seen multiple situations where people have merrily assumed that all drivers are normally distributed and hence can be modeled as such. In most events in nature, heights and weights of human beings, sizes of stars, it is fair to expect and find distributions that are normal or even close to normal. However, not so with business data. Because of the influence of human beings, business data tends to get pretty severely attenuated at places and stretched out at some other places. Now, there are a number of other important distributions to consider (which will probably form part of another post sometime), but assuming all distributions are normal is pure bunkum. But this is usually a rookie mistake! Move on to ...
2. Ignoring the probabilities of extreme tail events
Another quirk of business events is the size and frequency of tail events. Tail events astound us frequently with both their size and their frequency. Just when you thought Q4 08's GDP drop of close to 6% is a once-a-100-years event, it then goes and repeats itself in the next quarter. Ergo, with 10% falls in market cap in a day. Guess what you see the next trading day! Short advise is, be very afraid of things that happen in the tails. Because these events occur so infrequently, distributions are usually misleading in this space. So if you are expecting your model to tell you when things go bump at night, you will be in for a rude shock when they actually go bump. But why go to the tails when there are bigger things that lurk in the main body of the distribution, such as...
3. Assuming that model inputs are independent
Again, this is another example of a lazy assumption. People make these assumptions because they are obsessed with the tool at hand and its coolness-coefficient and cannot be bothered to use their heads and use the tool to solve the problem at hand. I am going to have a pretty big piece on lazy assumptions soon. (One of my favourite soap-box items!) When people run Monte Carlo simulations, the assumptions and inputs to the model are usually correlated to each other to different degrees. This means that the distributions of outcomes that you get at the end are going to crunched together (probability-density wise) at some places and are going to be sparse at some other places. But assuming a perfectly even distributions on either side of the mean is really not the goal here. The goal is to get as close an approximation of real-life distributions as possible. But then if only things were that simple! Now, you could be really smart and get all of the above just right and build a really cool tool. You could then get into the fourth fallacy of thinking ...
4. That it is about the distribution or the tool, it is NOT! It is about what you do with the results of the analysis
The Monte Carlo simulation tool is indeed just that, a tool. The distributions produced at the end of running the tool are not an end in themselves, they are an aid to decision making. In my experience, a well-thought out decision making framework needs to be created to make use of the distribution outputs. The decision-making framework could go something as follows. Let's take a framework to evaluate investment decisions, that uses NPV. One framework could be: I will make the investment only if a.) the mean NPV I can make is positive, and b.) less than 20% of the outcomes are negative NPV, and c.) less than 5% of the outcomes are negative NPV of less than $50 million. There's really no great science in coming up with these frameworks, but it has to be something that the decision maker is comfortable with and it should address uncertainty in outcomes.
So, have you come across some of these fallacies in your work? How have you seen the Monte Carlo tool used and misused in your work? And what decision making frameworks (if any) were allied with this tool to drive good decisions?
So, (drumroll), top Monte Carlo simulation fallacies I have come across.
1. Assuming all of the model drivers are normally distributed
Usually the biggest fallacy of them all. I have seen multiple situations where people have merrily assumed that all drivers are normally distributed and hence can be modeled as such. In most events in nature, heights and weights of human beings, sizes of stars, it is fair to expect and find distributions that are normal or even close to normal. However, not so with business data. Because of the influence of human beings, business data tends to get pretty severely attenuated at places and stretched out at some other places. Now, there are a number of other important distributions to consider (which will probably form part of another post sometime), but assuming all distributions are normal is pure bunkum. But this is usually a rookie mistake! Move on to ...
2. Ignoring the probabilities of extreme tail events
Another quirk of business events is the size and frequency of tail events. Tail events astound us frequently with both their size and their frequency. Just when you thought Q4 08's GDP drop of close to 6% is a once-a-100-years event, it then goes and repeats itself in the next quarter. Ergo, with 10% falls in market cap in a day. Guess what you see the next trading day! Short advise is, be very afraid of things that happen in the tails. Because these events occur so infrequently, distributions are usually misleading in this space. So if you are expecting your model to tell you when things go bump at night, you will be in for a rude shock when they actually go bump. But why go to the tails when there are bigger things that lurk in the main body of the distribution, such as...
3. Assuming that model inputs are independent
Again, this is another example of a lazy assumption. People make these assumptions because they are obsessed with the tool at hand and its coolness-coefficient and cannot be bothered to use their heads and use the tool to solve the problem at hand. I am going to have a pretty big piece on lazy assumptions soon. (One of my favourite soap-box items!) When people run Monte Carlo simulations, the assumptions and inputs to the model are usually correlated to each other to different degrees. This means that the distributions of outcomes that you get at the end are going to crunched together (probability-density wise) at some places and are going to be sparse at some other places. But assuming a perfectly even distributions on either side of the mean is really not the goal here. The goal is to get as close an approximation of real-life distributions as possible. But then if only things were that simple! Now, you could be really smart and get all of the above just right and build a really cool tool. You could then get into the fourth fallacy of thinking ...
4. That it is about the distribution or the tool, it is NOT! It is about what you do with the results of the analysis
The Monte Carlo simulation tool is indeed just that, a tool. The distributions produced at the end of running the tool are not an end in themselves, they are an aid to decision making. In my experience, a well-thought out decision making framework needs to be created to make use of the distribution outputs. The decision-making framework could go something as follows. Let's take a framework to evaluate investment decisions, that uses NPV. One framework could be: I will make the investment only if a.) the mean NPV I can make is positive, and b.) less than 20% of the outcomes are negative NPV, and c.) less than 5% of the outcomes are negative NPV of less than $50 million. There's really no great science in coming up with these frameworks, but it has to be something that the decision maker is comfortable with and it should address uncertainty in outcomes.
So, have you come across some of these fallacies in your work? How have you seen the Monte Carlo tool used and misused in your work? And what decision making frameworks (if any) were allied with this tool to drive good decisions?
Labels:
Decision Making,
Modeling,
Simulations,
Stress Testing
Wednesday, June 17, 2009
Why sitting down and talking does not help - (and what it means for the data scientist)
What is the sane person's advise to two people who cannot agree on something? It is usually sit down and resolve their differences. A set of recent recent studies seem to suggest that it doesn't really help.
Some recent studies have shown that when people with strong opposing positions are put together to talk it out, it makes them even more entrenched in their opinions. This is the point put forward by Cass Sunstein in his book Going to Extremes. Even when the groups/ people with opposing views are presented objective evidence, people tend to "see" what they want to believe in the data and ignore the rest.
Another study cited by the Economist struck a similar message. The study was looking at self-help books which stress positive thinking, and their impacts on people. What the study found was that positive thinking only helps for people who are predisposed to thinking positively. The study can be credited to Joanne Wood of the University of Waterloo in Canada and her colleagues. The researchers report in Psychological Science journal that when people with high self-esteem are made to repeat positive reinforcing messages, they do tend to take more positive positions (on standardized tests) than people who do not repeat positive reinforcing messages.
So far so good. It sounds as though positive reinforcing helps. But when the test was done on people with low self-esteem, the results were quite the opposite. People who repeated the positive reinforcing message took less positive positions than the ones that did not repeat the message. So it seems to imply that positive reinforcement actually hurts when applied to people who are inclined to believe otherwise. For me, this sounds like another example of people entrenching towards their own biases. When people with entrenched positions are forced to take a contrary position (or look at objective data), they tend to entrench even further on their original positions.
So what are the implications for the data scientist from all of this?
Mostly that predisposed positions produce a sort of "blindness" to objective data. We all suffer from confirmation bias, we like to believe what we like to believe. It is therefore a great effort for us to actively look at data objectively and take what that data is telling us, vs. putting the appropriate spin that suits us. The data scientist needs to exercise tremendous discipline here. It takes a superhuman effort not to succumb to our biases and to (not) believe what we want to believe, and take a genuine interest in forming an objective opinion.
One of the bigger learning for me in all of this is also the importance of give-and-take in making progress on any issue. Because of the entrenchment bias, people seldom change their views (i.e., come around to your way of thinking) based on objective data and logical persuasion. They only come along when they have skin in the game and for that to happen, there has to be an active element of give-and-take between the two parties. Which makes me even more admiring of GOOD politicians and diplomats. Their ability to keep moving forward on an issue in a bipartisan manner comes out of their skill in give-and-take, and thereby overcoming the entrenchment bias.
Some recent studies have shown that when people with strong opposing positions are put together to talk it out, it makes them even more entrenched in their opinions. This is the point put forward by Cass Sunstein in his book Going to Extremes. Even when the groups/ people with opposing views are presented objective evidence, people tend to "see" what they want to believe in the data and ignore the rest.
Another study cited by the Economist struck a similar message. The study was looking at self-help books which stress positive thinking, and their impacts on people. What the study found was that positive thinking only helps for people who are predisposed to thinking positively. The study can be credited to Joanne Wood of the University of Waterloo in Canada and her colleagues. The researchers report in Psychological Science journal that when people with high self-esteem are made to repeat positive reinforcing messages, they do tend to take more positive positions (on standardized tests) than people who do not repeat positive reinforcing messages.
So far so good. It sounds as though positive reinforcing helps. But when the test was done on people with low self-esteem, the results were quite the opposite. People who repeated the positive reinforcing message took less positive positions than the ones that did not repeat the message. So it seems to imply that positive reinforcement actually hurts when applied to people who are inclined to believe otherwise. For me, this sounds like another example of people entrenching towards their own biases. When people with entrenched positions are forced to take a contrary position (or look at objective data), they tend to entrench even further on their original positions.
So what are the implications for the data scientist from all of this?
Mostly that predisposed positions produce a sort of "blindness" to objective data. We all suffer from confirmation bias, we like to believe what we like to believe. It is therefore a great effort for us to actively look at data objectively and take what that data is telling us, vs. putting the appropriate spin that suits us. The data scientist needs to exercise tremendous discipline here. It takes a superhuman effort not to succumb to our biases and to (not) believe what we want to believe, and take a genuine interest in forming an objective opinion.
One of the bigger learning for me in all of this is also the importance of give-and-take in making progress on any issue. Because of the entrenchment bias, people seldom change their views (i.e., come around to your way of thinking) based on objective data and logical persuasion. They only come along when they have skin in the game and for that to happen, there has to be an active element of give-and-take between the two parties. Which makes me even more admiring of GOOD politicians and diplomats. Their ability to keep moving forward on an issue in a bipartisan manner comes out of their skill in give-and-take, and thereby overcoming the entrenchment bias.
Sunday, June 14, 2009
Connecting to the data centers - Netbooks
An apt follow-up to the post on data centers should be on the evolving tools to access the data centers. I had gone to Costco this morning and came across Netbooks. These are just stripped down laptops (or notebooks, if you may) that are perfect for accessing the Internet and getting your work done. Quite the rage with the college crowd apparently.
This is an attempt by the computer hardware industry to break the price barrier on portable computers. Used to about $1500
and came down to about $1000 four to five years back. But then the barrier stayed there for a while, with manufacturers adding feature on top of feature but refusing to reduce price. Till netbooks came along. These devices are priced at about $200-$350 and are pretty minimalist in their design. They have a fairly robust processor, a good sized keyboard and screen. No CD drive, for only neanderthals use a CD. But loaded when it comes to things like a Webcam, WiMax, etc. The netbook idea has two parallel phenomena that drove its evolution. One, the high-profile $100 laptop for third-world kids that really didn't go anywhere. The other was the increase in broadband penetration in the United States.
Another driver (probably) is the coming of age of the Millennial generation. When I grew up, the cool computer company of our times was Microsoft (or Apply, if you hated Microsoft). Both these companies had built their business models on paid products, products that needed upgrade and which cost money. We had therefore a certain reverence towards these companies and therefore an implicit acceptance of their pay-for-use business model.
Today's generation has come of age in the age of Google, Linux, Napster and other social networking sites. All of which are free. Today's kids feel less beholden to the idea of a computer company putting out formal products which you need to pay for and which get upgraded once every two years, for which you need to pay for again. In today's age, the idea of freeware and products that actively evolve with use is becoming more and more accepted. Ergo, the netbook.
Enough of my pop-psychology for now. Anyway, netbooks are really cool gadgets and I am tempted to get one really soon. The Economist had a good article on the subject. Let me know if you are early adopters of netbooks and your experiences so far.
This is an attempt by the computer hardware industry to break the price barrier on portable computers. Used to about $1500

Another driver (probably) is the coming of age of the Millennial generation. When I grew up, the cool computer company of our times was Microsoft (or Apply, if you hated Microsoft). Both these companies had built their business models on paid products, products that needed upgrade and which cost money. We had therefore a certain reverence towards these companies and therefore an implicit acceptance of their pay-for-use business model.
Today's generation has come of age in the age of Google, Linux, Napster and other social networking sites. All of which are free. Today's kids feel less beholden to the idea of a computer company putting out formal products which you need to pay for and which get upgraded once every two years, for which you need to pay for again. In today's age, the idea of freeware and products that actively evolve with use is becoming more and more accepted. Ergo, the netbook.
Enough of my pop-psychology for now. Anyway, netbooks are really cool gadgets and I am tempted to get one really soon. The Economist had a good article on the subject. Let me know if you are early adopters of netbooks and your experiences so far.
Friday, June 12, 2009
Stress testing your model - Part 3/3
We discussed two techniques of ensuring the robustness of models in two previous posts. In the first post, we discussed out-of-sample validation. In the second post, we discussed sensitivity analysis. I find sensitivity analysis to be a really valuable technique for ensuring the robustness of model outputs and decisions driven by models - but only when it is done right.
Another and a more computing-intensive technique of ensuring model output robustness is Monte Carlo simulation. Monte Carlo simulation basically involves running the models literally thousands of time and changing each of the inputs a little with every run. With advances in computing power and the power being within reach of most modelers and researchers, it has become fairly easy to set up and run the simulation.
So let's say, we have a model with 3 inputs. And now let's assume that the inputs are varied in 10 steps over its entire valid range. So now the model will produce 1000 different outputs for various values of inputs (1000 = 10 x 10 x 10), each output having a theoretical probability of 0.001.
How are the inputs varied?
Typically using a distribution that varies the inputs in a probabilistic manner. The input distribution is the most important assumption that goes into the Monte Carlo simulation. The typical approach is to assume that most events are normally distributed. But the reality is that normal distribution is usually observed only in natural phenomena. In most business applications, distributions are usually skewed in one direction. (Take loan sizes on a financial services product, like a credit card. The distribution is always skewed towards the higher side, as balances cannot be less than zero but can take really large positive values.)
Correlation or covariance of the inputs
In a typical business model, inputs are seldom independent; they have various degrees of correlation. It is important to keep this correlation in mind while running the scenarios. By factoring in covariance of inputs explicitly while running the simulation, the output is probabilistically weighted towards results which occur when the inputs are correlated.
Of course, as with any piece of modeling, there are ways in which this technique can be misused. Some of my pet gripes about MC simulation will form the subject of a later post.
Another and a more computing-intensive technique of ensuring model output robustness is Monte Carlo simulation. Monte Carlo simulation basically involves running the models literally thousands of time and changing each of the inputs a little with every run. With advances in computing power and the power being within reach of most modelers and researchers, it has become fairly easy to set up and run the simulation.
So let's say, we have a model with 3 inputs. And now let's assume that the inputs are varied in 10 steps over its entire valid range. So now the model will produce 1000 different outputs for various values of inputs (1000 = 10 x 10 x 10), each output having a theoretical probability of 0.001.
How are the inputs varied?
Typically using a distribution that varies the inputs in a probabilistic manner. The input distribution is the most important assumption that goes into the Monte Carlo simulation. The typical approach is to assume that most events are normally distributed. But the reality is that normal distribution is usually observed only in natural phenomena. In most business applications, distributions are usually skewed in one direction. (Take loan sizes on a financial services product, like a credit card. The distribution is always skewed towards the higher side, as balances cannot be less than zero but can take really large positive values.)
Correlation or covariance of the inputs
In a typical business model, inputs are seldom independent; they have various degrees of correlation. It is important to keep this correlation in mind while running the scenarios. By factoring in covariance of inputs explicitly while running the simulation, the output is probabilistically weighted towards results which occur when the inputs are correlated.
Of course, as with any piece of modeling, there are ways in which this technique can be misused. Some of my pet gripes about MC simulation will form the subject of a later post.
Wednesday, June 10, 2009
The massive cloud of 1s and 0s
Read an interesting article in the New York Times about the growth in data centers as we become an increasingly internet based world. Some of the numbers aroun
d the data center capacities at places like Microsoft, Google and for e-commerce or bidding sites like Amazon and eBay, was quite astounding. Not to mention the various electronic financial exchanges in the world.
Some of the numbers are quite astounding. Microsoft has more than 200,000 and its massively bigger competitor has to have more. And this massive data center infrastructure capability is already beginning to have a serious impact on the power requirements for our new world. More power for our data centers to upload of pictures of the weekend do onto Facebook, or an intact polar ice-cap. You chose!

Some of the numbers are quite astounding. Microsoft has more than 200,000 and its massively bigger competitor has to have more. And this massive data center infrastructure capability is already beginning to have a serious impact on the power requirements for our new world. More power for our data centers to upload of pictures of the weekend do onto Facebook, or an intact polar ice-cap. You chose!
Labels:
Computing,
Computing Infrastructure,
Data Centers
Tuesday, June 9, 2009
Green shoots ... or bust
Equity markets, both international and US, seem to have taken to heart the signs of bottoming of the world economy and the rebound seen in Asia. The Brazilian, Chinese and Indian stock markets are at least 50% higher than the lows in Q4 2008. The DJIA went down to the 6500 for a while but has since rebounded to gyrating around the 8500 market and has on occasion, flirted with the 9000 level.
The rates of job-loss is falling in the US and despite a glut in world oil supply, crude prices are nearing the $70 mark after crashing down to the $30s only recently. Are we past the worst then? Despite the lingering weakness in W.Europe (something seen arguably since the start of WW II!), the signs of economic recovery seem to be unmistakable.
Is the US consumer then going to go back to his/her free-spending ways? While we seem to have come up a fair bit from the Q4 depths, at least from a consumer confidence standpoint, there could be a few big obstacles to growth.
1. The budget deficit. With the famous American aversion to taxes and the growing burden of entitlements (driven mainly by healthcare costs) as the baby-boomer generation retires, the deficit is only going to get worse.
2. The cost of borrowing to feed the deficit. The US Treasury's place of pride as the investment of the highest quality could be under threat as the domestic debt as a % of GDP grows. The US government will need to increasingly borrow more and pay higher interest rates for the borrowing. The higher interest burden is going to crimp the ability to make productive investments.
3. Finally, with increasing protectionism and government involvement in the economy, the vitality of US business enterprise to identify and capitalize on opportunity looks to be suppressed for the next several years.
A number of prognosticators have made some long-range predictions of the US Economy in this article. An interesting read, as the forecasters have taken a 5-10 year view rather than the 6-12 month view typically taken by realtor types. Another interesting article, about the long term speed limit of the US economy from the Economist. Summarizing the article,
According to Robert Gordon, a productivity guru at Northwestern University, America’s trend rate of growth in 2008 was only 2.5%, the lowest rate in its history, and well below the 3-3.5% that many took for granted a few years ago. Without factoring in the financial crisis, Mr Gordon expects potential growth to fall to 2.35% over the coming years.
The rates of job-loss is falling in the US and despite a glut in world oil supply, crude prices are nearing the $70 mark after crashing down to the $30s only recently. Are we past the worst then? Despite the lingering weakness in W.Europe (something seen arguably since the start of WW II!), the signs of economic recovery seem to be unmistakable.
Is the US consumer then going to go back to his/her free-spending ways? While we seem to have come up a fair bit from the Q4 depths, at least from a consumer confidence standpoint, there could be a few big obstacles to growth.
1. The budget deficit. With the famous American aversion to taxes and the growing burden of entitlements (driven mainly by healthcare costs) as the baby-boomer generation retires, the deficit is only going to get worse.
2. The cost of borrowing to feed the deficit. The US Treasury's place of pride as the investment of the highest quality could be under threat as the domestic debt as a % of GDP grows. The US government will need to increasingly borrow more and pay higher interest rates for the borrowing. The higher interest burden is going to crimp the ability to make productive investments.
3. Finally, with increasing protectionism and government involvement in the economy, the vitality of US business enterprise to identify and capitalize on opportunity looks to be suppressed for the next several years.
A number of prognosticators have made some long-range predictions of the US Economy in this article. An interesting read, as the forecasters have taken a 5-10 year view rather than the 6-12 month view typically taken by realtor types. Another interesting article, about the long term speed limit of the US economy from the Economist. Summarizing the article,
According to Robert Gordon, a productivity guru at Northwestern University, America’s trend rate of growth in 2008 was only 2.5%, the lowest rate in its history, and well below the 3-3.5% that many took for granted a few years ago. Without factoring in the financial crisis, Mr Gordon expects potential growth to fall to 2.35% over the coming years.
Sunday, June 7, 2009
Stress testing your model - Part 2/3
Continuing on the topic of risk management for models. After building a model, how do you make sure the model remains robust under working conditions? More importantly, make sure it works well under extreme conditions? We discussed the importance of independent validation for empirical models in a previous post. In my experience, model failures have been frequent when the validation process has been superficial.
Now, I want to move on to sensitivity analysis. Sensitivity analysis involves understanding the variability of the model output as the inputs to the model are varied. The inputs are changed by + or - 10 to 50% and the output is recorded. The range of outputs gives a sense of the various outcomes that can be anticipated and one needs to prepare for. Sensitivity analysis can also be used to stress test the other components of the system which the model drives. For example, let's say the output of the model is a financial forecast that goes into a system that is used to drive, deposit generation. The sensitivity analysis output gives an opportunity to check the robustness of the downstream system. By knowing that one might require occasionally to generate deposits at 4-5 times the usual monthly volumes, one can prepare accordingly.
Now, sensitivity analysis is one piece of stress testing that has usually been misdirected and incomplete. Good sensitivity analysis looks at both the structural components of the model as well as the inputs to the model. Most sensitivity analysis I have encountered stress only the structural components. What is the difference between the two?
Let's say, you have a model to project the performance of the balance sheet of a bank. One of the typical stresses that one would apply is to the expected level of losses on the loan portfolio of the bank. A stress of 20-50% and sometimes even 100% increase in losses is applied and the model outputs are assessed. When this is done consistently with all the other components of the balance sheet, you can get a sense of the sensitivity of the model to various components.
But that's not the same as the sensitivity to inputs. Because inputs are based in real-world phenomena, their impact is usually spread out to multiple components in the model. For example, if the 100% increase in losses were driven by a recession in the economy, there would be other impacts that one would need to worry about. Now, a recession is usually accompanied by a flight to quality from investors. So if there is a recessionary outlook, the value of equity holdings could crash as well due to equity investors moving out from equities (selling) and into more stable instruments. A third impact could be the impact of higher capital requirements on the value of traded securities . As other banks face the same recessionary environment, their losses could increase to such an extent that a call to increase capital becomes inevitable. How does one increase capital? The easiest route is to liquidate existing holdings. Driving a greater fall in the market prices of traded securities. Thus, putting further stresses on the balance sheet.
So, the scenario of running a 50% increase in loan losses is a purely illusory one. When loan losses increase, one has to contend with what the fundamental driver could be and how can that fundamental driver impact other portions of the balance sheet.
The other place where sensitivity analysis is often incomplete is by not looking at the impact of upstream and downstream processes and strategies. A model is never a stand-alone entity. It has upstream sources of data and down-stream uses of the model output. So if the model has to face situations where there are extreme values of inputs, what could be the implications on upstream and downstream strategies? These are the questions any serious model builder should be asking.
This discussion on sensitivity analysis has hopefully been eye-opening to modeling practitioners. Now, we will go on to a third technique, Monte Carlo simulation in another post. But before we go there, what are other examples of sensitivity analyses that you have seen in your work? How has this analysis been used effectively (or otherwise)? What are good graphical ways of sharing the output?
Now, I want to move on to sensitivity analysis. Sensitivity analysis involves understanding the variability of the model output as the inputs to the model are varied. The inputs are changed by + or - 10 to 50% and the output is recorded. The range of outputs gives a sense of the various outcomes that can be anticipated and one needs to prepare for. Sensitivity analysis can also be used to stress test the other components of the system which the model drives. For example, let's say the output of the model is a financial forecast that goes into a system that is used to drive, deposit generation. The sensitivity analysis output gives an opportunity to check the robustness of the downstream system. By knowing that one might require occasionally to generate deposits at 4-5 times the usual monthly volumes, one can prepare accordingly.
Now, sensitivity analysis is one piece of stress testing that has usually been misdirected and incomplete. Good sensitivity analysis looks at both the structural components of the model as well as the inputs to the model. Most sensitivity analysis I have encountered stress only the structural components. What is the difference between the two?
Let's say, you have a model to project the performance of the balance sheet of a bank. One of the typical stresses that one would apply is to the expected level of losses on the loan portfolio of the bank. A stress of 20-50% and sometimes even 100% increase in losses is applied and the model outputs are assessed. When this is done consistently with all the other components of the balance sheet, you can get a sense of the sensitivity of the model to various components.
But that's not the same as the sensitivity to inputs. Because inputs are based in real-world phenomena, their impact is usually spread out to multiple components in the model. For example, if the 100% increase in losses were driven by a recession in the economy, there would be other impacts that one would need to worry about. Now, a recession is usually accompanied by a flight to quality from investors. So if there is a recessionary outlook, the value of equity holdings could crash as well due to equity investors moving out from equities (selling) and into more stable instruments. A third impact could be the impact of higher capital requirements on the value of traded securities . As other banks face the same recessionary environment, their losses could increase to such an extent that a call to increase capital becomes inevitable. How does one increase capital? The easiest route is to liquidate existing holdings. Driving a greater fall in the market prices of traded securities. Thus, putting further stresses on the balance sheet.
So, the scenario of running a 50% increase in loan losses is a purely illusory one. When loan losses increase, one has to contend with what the fundamental driver could be and how can that fundamental driver impact other portions of the balance sheet.
The other place where sensitivity analysis is often incomplete is by not looking at the impact of upstream and downstream processes and strategies. A model is never a stand-alone entity. It has upstream sources of data and down-stream uses of the model output. So if the model has to face situations where there are extreme values of inputs, what could be the implications on upstream and downstream strategies? These are the questions any serious model builder should be asking.
This discussion on sensitivity analysis has hopefully been eye-opening to modeling practitioners. Now, we will go on to a third technique, Monte Carlo simulation in another post. But before we go there, what are other examples of sensitivity analyses that you have seen in your work? How has this analysis been used effectively (or otherwise)? What are good graphical ways of sharing the output?
Saturday, June 6, 2009
Stress testing your model - Part 1/3
So, you've built a model. You have been careful about understanding your data, transforming it appropriately, used the right modeling technique, done an independent validation (if it is an empirical model) and now you are ready to use the model to make forecasts, drive decisions, etc.
Wait. Not so fast. Before the model is ready for prime time, you need to make sure that the model is robust. What defines a robust model?
- the inputs should cover not just the expected events but also extreme events
- the model should not break down (i.e., mispredict) when the inputs turn extreme (Well, no model can be expected to perform superbly when the inputs turn extreme. If models could do that, the events wouldn't be termed extreme events. But the worst thing that a model can do is provide an illusion of normal (english usage) outputs when the inputs are extreme.
I want to share some of the techniques that are used for understanding the robustness of the models, what I like about them and what I don't.
1. When it comes to empirical models, one of the most useful techniques is Out of Sample Validation. This is done by building the model on one data set and validating the algorithm on another. For the truest validation, the validation dataset should be independent of the build, should be drawn from a different time period. "Check-the-box" type validation is when you validate the model on a portion of the build sample itself. Such validation often holds and just as often offers a false sense of security, because in real terms, you have really not validated anything.
Caveat: Out of sample validation is of no use if the future is going to look very different from the past. Validating a model to predict the probability of mortgage default using conventional mortgages data would have been of no use in a world where no-documentation mortgages and other exotic-term mortgages were being marketed.
The other two approaches I want to discuss are Sensitivity Analysis and Monte Carlo Simulation. I will cover them in subsequent posts.
Wait. Not so fast. Before the model is ready for prime time, you need to make sure that the model is robust. What defines a robust model?
- the inputs should cover not just the expected events but also extreme events
- the model should not break down (i.e., mispredict) when the inputs turn extreme (Well, no model can be expected to perform superbly when the inputs turn extreme. If models could do that, the events wouldn't be termed extreme events. But the worst thing that a model can do is provide an illusion of normal (english usage) outputs when the inputs are extreme.
I want to share some of the techniques that are used for understanding the robustness of the models, what I like about them and what I don't.
1. When it comes to empirical models, one of the most useful techniques is Out of Sample Validation. This is done by building the model on one data set and validating the algorithm on another. For the truest validation, the validation dataset should be independent of the build, should be drawn from a different time period. "Check-the-box" type validation is when you validate the model on a portion of the build sample itself. Such validation often holds and just as often offers a false sense of security, because in real terms, you have really not validated anything.
Caveat: Out of sample validation is of no use if the future is going to look very different from the past. Validating a model to predict the probability of mortgage default using conventional mortgages data would have been of no use in a world where no-documentation mortgages and other exotic-term mortgages were being marketed.
The other two approaches I want to discuss are Sensitivity Analysis and Monte Carlo Simulation. I will cover them in subsequent posts.
Tuesday, June 2, 2009
Using your grey cells ...
Growing up as a singularly unathletic child, my favourite form of recreation was usually through books. And a favourite amongst the books were Agatha Christie's murder mysteries featuring Hercule Poirot. Poirot fascinated me. I guess there was the element of vicariously living through the act of evil being punished by good. (Which probably attracts us to all mystery writers).
But another aspect that made Poirot more appealing than the more energetic specimens like Sherlock Holmes was his reliance on "ze little grey cells". The power of analytical reasoning practiced through the simple mechanism of question and answer being used to solve fiendishly difficult murders. How romantic an idea!
I recently came across a intriguing set of problems, which require rigorous exercise of the grey cells. Called Fermi problems, these are just plain old estimation problems. Typical examples go like "estimate the number of piano tuners in New York City", "estimate the number of Mustangs in the US". It requires one to start out with some basic facts and figures and then get to the answer through a number of logical reasoning steps. For the piano tuner question, it is usually good to start off with some estimate of the population of New York City. Starting off with ridiculous numbers (like 1 million or 100 million) will definitely lead you to the wrong answer. So, what the estimation exercise really calls for is some general knowledge with some ability to think and reason logically.
The solution to the piano tuner problem typically goes as follows:
- Number of people in NYC -> Number of households
- Number of households -> Estimating the numbers with a piano
- Number of pianos -> Some tuning frequency -> Demand for number of pianos that need tuning in a month
- Assuming a certain number of pianos that can be tuned in a day and a certain number of working days, you get to the number of likely tuners
The exercise definitely teaches some ability to make logical connections. The other thing this type of thinking teaches is parsimony of assumptions. One could make the problem more complex by assuming a different population for NYC's different buroughs, different estimates for the proportion of households with pianos for Manhattan vs the Bronx and so on. In practice however, these assumptions only introduce false precision to the answer. Just because you have thought through the solution in an enormous degree of detail doesn't necessarily make it right.
Some typical example of Fermi problems can be found at this link. Enjoy the experience. And I would love to hear some of the feelings that strike you as you try and solve these problems. Some of the "a-ha" moments for me were around the parsimony of assumptions, needing to find the point of greatest uncertainty and then fix it with the least cost, so as to narrow down the range of answers.
The exercise overall taught me a fair bit about about modeling, the way we approach modeling problems, ranges of uncertainty and how we deal with them, parsimony of assumptions.
But another aspect that made Poirot more appealing than the more energetic specimens like Sherlock Holmes was his reliance on "ze little grey cells". The power of analytical reasoning practiced through the simple mechanism of question and answer being used to solve fiendishly difficult murders. How romantic an idea!
I recently came across a intriguing set of problems, which require rigorous exercise of the grey cells. Called Fermi problems, these are just plain old estimation problems. Typical examples go like "estimate the number of piano tuners in New York City", "estimate the number of Mustangs in the US". It requires one to start out with some basic facts and figures and then get to the answer through a number of logical reasoning steps. For the piano tuner question, it is usually good to start off with some estimate of the population of New York City. Starting off with ridiculous numbers (like 1 million or 100 million) will definitely lead you to the wrong answer. So, what the estimation exercise really calls for is some general knowledge with some ability to think and reason logically.
The solution to the piano tuner problem typically goes as follows:
- Number of people in NYC -> Number of households
- Number of households -> Estimating the numbers with a piano
- Number of pianos -> Some tuning frequency -> Demand for number of pianos that need tuning in a month
- Assuming a certain number of pianos that can be tuned in a day and a certain number of working days, you get to the number of likely tuners
The exercise definitely teaches some ability to make logical connections. The other thing this type of thinking teaches is parsimony of assumptions. One could make the problem more complex by assuming a different population for NYC's different buroughs, different estimates for the proportion of households with pianos for Manhattan vs the Bronx and so on. In practice however, these assumptions only introduce false precision to the answer. Just because you have thought through the solution in an enormous degree of detail doesn't necessarily make it right.
Some typical example of Fermi problems can be found at this link. Enjoy the experience. And I would love to hear some of the feelings that strike you as you try and solve these problems. Some of the "a-ha" moments for me were around the parsimony of assumptions, needing to find the point of greatest uncertainty and then fix it with the least cost, so as to narrow down the range of answers.
The exercise overall taught me a fair bit about about modeling, the way we approach modeling problems, ranges of uncertainty and how we deal with them, parsimony of assumptions.
Sunday, May 31, 2009
The counter-intuitiveness of probability - small sample sizes
Sports enthusiasts amongst you (and who read this website) have to be into sports statistics. My earliest memories about cricket were not about me playing my first impressive air cover-drive or about charging in (in my shorts) and delivering a toe-crushing yorker fired towards the base of the leg-stump. My most vivid early memories were about buying cricket and sports magazines hot off the presses and reading sheets and sheets of cricket statistics.
These statistics covered a wide range of topics. There was the usual highest number of runs, highest number of wickets type stuff. There were also ratio-type statistics: number of 5WI per game, number of centuries per innings, proportion of winning games in which a certain batsman scored a century. With a lot of the ratio metrics, there was usually a minimum in the form of number of matches, innings the player should have played before being part of the statistics. For my unschooled mind, it was a way of eliminating the one-match wonders, the flukes from the more serious practitioners.
With the gift of recapitulating some of those memories and looking at them afresh with my relatively better schooled analytical mind, it struck me that what the statistician (or more precisely, compiler of statistics) was trying to do was to use the law of large numbers (and large event sizes) to produce a distribution centered around the true mean. Put in another way, when the sample is small, one is more apt to get extreme values. So, if the "true" average for number of innings per century is 4.5, there could be 3-4 innings' stretches where the player scores consecutive hundreds, pushing the average well down. And if these stretches occur (by chance) at the start of someone's career, it is wont to lead to wrong conclusions about the ability of the batsman.
A simple exercise. How many Heads to expect out of 5 coin tosses with an unbiased coin? One would say, between 2 and 3 Heads. But if you had one trial vs several, what would you expect? What would the mean look like and what would the distribution be? For the sake of simplicity, let's label 2 and 3 Heads as Central values, 1 and 4 as Off-Central and 0 and 5 as Extreme values.
I did a quick simulation. Following are the results around mean, Central, Off-Central and Extreme values.
With 1 trial, the results were: 3,1,0,0.
With 3 trials, the results were: 1.66,0,2,1.
With 5 trials, the results were: 1.8,1,4,0.
With 10 trials, the results were: 2.7,10,0,0.
Now 10 is no magic number but it is easy to see how one can get a greater proportion of central values (or values closer to the mean) as the number of trials gets larger. I would love to get a cool snip of SAS or R code that can do this simulation. And hence the push to eliminate outliers by increasing the number of trials.
Now this is the paradox of small trials. When the number of trials are small, when you have fewer shots at observing something, chances are greater that you'd actually see more extreme values whose frequency cannot be predicted. What does this mean for risk management? Does one try and manage greater volatility at a unit level or lesser at the system level? And how do you make sure the greater volatility at the unit level does not sink your business?
These statistics covered a wide range of topics. There was the usual highest number of runs, highest number of wickets type stuff. There were also ratio-type statistics: number of 5WI per game, number of centuries per innings, proportion of winning games in which a certain batsman scored a century. With a lot of the ratio metrics, there was usually a minimum in the form of number of matches, innings the player should have played before being part of the statistics. For my unschooled mind, it was a way of eliminating the one-match wonders, the flukes from the more serious practitioners.
With the gift of recapitulating some of those memories and looking at them afresh with my relatively better schooled analytical mind, it struck me that what the statistician (or more precisely, compiler of statistics) was trying to do was to use the law of large numbers (and large event sizes) to produce a distribution centered around the true mean. Put in another way, when the sample is small, one is more apt to get extreme values. So, if the "true" average for number of innings per century is 4.5, there could be 3-4 innings' stretches where the player scores consecutive hundreds, pushing the average well down. And if these stretches occur (by chance) at the start of someone's career, it is wont to lead to wrong conclusions about the ability of the batsman.
A simple exercise. How many Heads to expect out of 5 coin tosses with an unbiased coin? One would say, between 2 and 3 Heads. But if you had one trial vs several, what would you expect? What would the mean look like and what would the distribution be? For the sake of simplicity, let's label 2 and 3 Heads as Central values, 1 and 4 as Off-Central and 0 and 5 as Extreme values.
I did a quick simulation. Following are the results around mean, Central, Off-Central and Extreme values.
With 1 trial, the results were: 3,1,0,0.
With 3 trials, the results were: 1.66,0,2,1.
With 5 trials, the results were: 1.8,1,4,0.
With 10 trials, the results were: 2.7,10,0,0.
Now 10 is no magic number but it is easy to see how one can get a greater proportion of central values (or values closer to the mean) as the number of trials gets larger. I would love to get a cool snip of SAS or R code that can do this simulation. And hence the push to eliminate outliers by increasing the number of trials.
Now this is the paradox of small trials. When the number of trials are small, when you have fewer shots at observing something, chances are greater that you'd actually see more extreme values whose frequency cannot be predicted. What does this mean for risk management? Does one try and manage greater volatility at a unit level or lesser at the system level? And how do you make sure the greater volatility at the unit level does not sink your business?
Labels:
Extreme Values,
Law of Large Numbers,
Probability
Subscribe to:
Posts (Atom)